Speech Recognition Technology: Applications, Algorithms, and Impact on AI
Explore the innovative applications of AI in speech recognition, transforming technology and communication like never before.
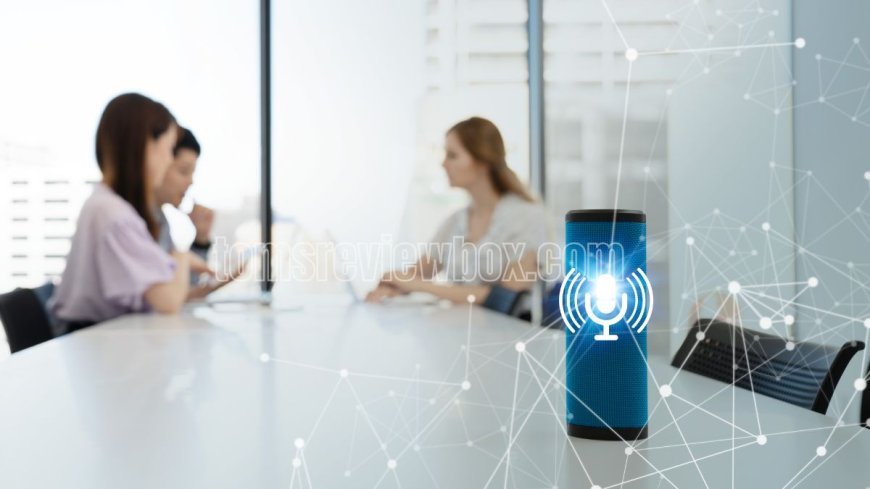
The impact of AI on speech recognition has completely changed the way we communicate with machines. Thanks to artificial intelligence (AI), it’s now possible to talk to devices and have them understand you. As a result, finding information, sending messages, and so on become quicker and more convenient!
AI algorithms learn by analyzing speech patterns, which leads to improved accuracy and efficiency over time, adapting to different accents and dialects. While consumers primarily benefit from this technology, companies and government agencies use it to improve customer service, reduce costs and increase efficiency.
As AI becomes more sophisticated, so too does the magic of speech recognition, bringing more actionable solutions to life across industries. Familiarity with these emerging best practices will set you up to capitalize on AI in health in accessible and impactful ways.
Key Takeaways
- AI-powered speech recognition technology is essential for enhancing user interaction with devices and improving accessibility for diverse groups. Learning what it really means and why it matters will help you better use these systems across multiple applications.
- It is through machine learning that these systems have become more accurate and efficient in recognizing speech. Understanding these technologies is key to making them work for you and your projects.
- As speech recognition technology has matured, it has moved from rudimentary systems to sophisticated AI-fueled models. Keeping up with these developments is key to being able to use the best solutions.
- various algorithms, including Deep Neural Networks, Convolutional Neural Networks Each algorithm has its strengths, which make them suitable for different SLR tasks. Test them out and determine their strengths to inform your next innovation endeavor. This will set you up to determine the appropriate solution for your unique requirements.
- Ongoing development and feedback from users are essential for improving speech recognition systems, continuing to work toward the goal of increased accessibility. Collaborating with users and integrating their feedback will result in a product that performs better and meets user needs.
- Addressing ethical considerations, including data privacy and accessibility, are key in advancing speech recognition development. Understanding these concerns will help you use AI in ways that are responsible and ethical.
Speech Recognition and AI: An Overview
AI-powered speech recognition is the backbone of the modern technology, allowing for a natural, conversational exchange between humans and machines. This deep learning technology enables applications and computers to comprehend and transcribe spoken language, revolutionizing the way users interact with technology.
Speech recognition is in a period of amazing flux, promise, and opportunity. It is expected to continue increasing at a compound annual growth rate (CAGR) of 17.2%, exceeding $26.8 billion in market value by 2025.
What is AI-Powered Speech Recognition?
AI-driven speech recognition systems leverage the use of acoustic models to examine sound. They further use large language models to convert those sounds into understandable text.
Machine learning makes it possible to improve precision by allowing systems to learn from massive amounts of data. This advancement makes it easier for the technology to learn and understand different accents and speech patterns.
This functionality provides for real-time processing, meaning applications can respond immediately to voice commands.
Significance of Speech Recognition in AI
By breaking down one of the last barriers to intuitive human-computer interaction, speech recognition drastically improves usability for all user demographics—most notably, user groups with disabilities.
This last feature has really changed the landscape of customer service, where AI is expected to manage 95% of all interactions by 2025. Additionally, inclination towards speech interfaces is strong, with customers starting 70% of self-service interactions via voice in 2023.
Evolution of Speech Recognition Technology
The evolution of speech recognition went from simple systems to highly complex AI-powered solutions. Today’s systems are much more powerful than their ancestors, having improved on past failures in accuracy and timing.
Changing the tools User feedback from all levels of expertise has been invaluable in department-wide efforts to improve these tools to better serve user needs.
How AI Powers Speech Recognition
Speech recognition technology, particularly through the use of AI speech recognition systems, is making it possible for computers to understand and process human speech with astounding accuracy. This powerful capability is made possible by crucial pieces like acoustic modeling and language modeling.
Acoustic Modeling Explained
The first step to make sense of a speech signal is acoustic modeling. Creating statistical models of sound waves in order to classify phonemes is essential. Machine learning techniques are a critical part of that process. They enhance models’ ability to learn from massive amounts of audio data and boost their speech recognition accuracy.
Higher accuracy in the acoustic models directly translates to better overall speech recognition performance.
Language Modeling Explained
At a more granular level, language modeling predicts sequences of words, which is crucial for effective speech recognition AI technology. It improves the understanding of context, allowing conversational AI systems to determine what words are most likely based on prior inputs. Building useful language models is no small task, especially when it comes to grasping the nuances and variations of human speech.
Feature Extraction Techniques
Among the most common key feature extraction techniques used in speech recognition AI technology are MFCCs and spectrogram analysis. These features enhance the capabilities of speech recognition systems by distinguishing speech sounds, focusing on informative qualities. By selecting the most predictive features, AI models can significantly improve their performance.
Decoding Process in Detail
During this phase, audio signals are decoded as they are transformed into text through various stages such as feature extraction and pattern recognition. Optimization methods such as dynamic programming and beam search intelligently search through potential sequences of words to produce the most accurate transcriptions.
The hurdles are still high — particularly when speaking in noisy places or with an accent.
Algorithms and Models in Speech Recognition
Speech recognition AI technology is rooted in the use of algorithms and models to accurately understand spoken language. Each speech recognition algorithm has a specific role, tackling different challenges associated with recognizing speech with high accuracy and computational speed. A solid understanding of these speech recognition solutions will go a long way towards effectively applying this technology across nearly every discipline.
1. Deep Neural Networks (DNNs) for Accuracy
DNNs have proven to dramatically improve performance on many speech recognition tasks, using high-level audio features to recognize phonemes, the most basic unit of sound. Their architecture allows them to understand detailed patterns in the data while showing their superiority over previously used models.
DNNs constantly improve their precision, which has made them ubiquitous in applications such as Google Assistant.
2. Convolutional Neural Networks (CNNs) for Noise
CNNs are particularly well-suited for filtering out background noise, an important element of making speech recognition function better in loud environments. First, they are excellent at handling specific types of data, like audio inputs.
This capability improves intelligibility and better isolates desired sounds, a major benefit in automotive VR environments.
3. Recurrent Neural Networks (RNNs) for Context
RNNs, as part of advanced speech recognition AI technology, are particularly well suited for modeling sequential information, enhancing the context understanding of spoken language, although they struggle with longer sequences compared to superior models like transformers.
4. Transformer Networks: A Modern Approach
Transformers transform the game joint in automatic speech recognition (ASR) due to their efficiency in processing long-range dependencies. Their out-of-the-box thinking has opened up big strides in natural language AI, empowering AI assistants to be more human-like and interactive.
5. Hybrid Models: Combining Strengths
Hybrid models combine different speech recognition algorithms, taking advantage of their respective strengths. Such a strategy can result in more powerful performance across various use cases, reflecting the growing expectations in the speech recognition ai technology industry.
Model Type |
Strengths |
Weaknesses |
---|---|---|
DNNs |
High accuracy |
Requires extensive data |
CNNs |
Noise handling |
Limited context awareness |
RNNs |
Context understanding |
Struggles with long sequences |
Transformers |
Excellent for long-range data |
Computationally intensive |
Hybrid Models |
Balanced performance |
Complexity in integration |
Applications Across Industries
Speech recognition technology, particularly through ai speech recognition systems, has touched nearly every industry, changing the way we communicate with devices and services. This innovation enhances user experience and provides meaningful value, making the technology a valuable tool across numerous industries.
Speech Recognition in Call Centers
In medical call centers, for instance, speech recognition increases productivity by automating repetitive activities. Real-time transcription improves customer experiences, giving contact center agents more time to help customers solve issues instead of jotting down notes.
Advanced sentiment analysis easily identifies positive or negative customer sentiment, allowing your customer service reps to respond in kind.
AI Speech Tech in Banking & Finance
Smart banking technology powered by speech recognition simplifies every banking transaction with just a few words. Voice authentication decreases fraud risk, making sure that only authorized users can access their accounts.
For example, banks use this AI-powered speech technology to help customers choose the right service, increasing positive satisfaction scores.
Speech Recognition in Telecommunications
Speech recognition is a huge benefit for telecommunications. It improves the overall service by adding voice commands that help direct users more easily through the app, especially useful for people with disabilities.
Mobile applications like Whatsapp, Telegram, and Signal incorporate this technology, keeping communication secure while letting users make calls or send messages seamlessly.
AI Voice Solutions in Healthcare
When it comes to the healthcare industry, speech recognition applications are a crucial component. They help with things like documentation and report generation, which saves professionals time.
Better communication tools help patients, too, making sure they get the information they need when they need it.
Speech Recognition in Media and Marketing
Speech recognition is at the heart of how media organizations are using AI in the creative industry. By analyzing consumer behavior at staggering speeds, it provides marketers the tools to better understand consumer preferences.
Voice search optimization is transforming the marketing landscape, opening doors for businesses and organizations to become more accessible to their future patrons.
Other Emerging Applications
Even newer fields such as education and smart homes are embracing the potential of speech recognition AI technology. Innovations in this area hold great promise to enhance our educational environments and the lives of our constituents while leveraging AI solutions to create new opportunities for tomorrow.
Overcoming Challenges in AI Speech Tech
While developing powerful speech recognition ai systems is a rewarding pursuit, it poses numerous challenges that can affect both precision and user experience, emphasizing the need for effective speech recognition solutions in their development.
Handling Accents and Dialects
Both accents and dialects are particularly heavy lifts for speech recognition. Differences in accent or dialect can create a great deal of confusion. That’s why training models on a wide representation of speech data is so important.
For example, adding the recordings of speakers from various ethnic backgrounds helps the model learn patterns in different speech styles. Technical solutions such as fine-tuning algorithms according to user feedback can help increase recognition accuracy across dialects.
Managing Background Noise
We know that background noise can severely impact speech recognition capabilities. Techniques like echo cancellation and adaptive filtering are essential in addressing this issue. While high-quality speech recognition algorithms play a crucial role in isolating the speaker’s voice from background noise, real-world robust training datasets that encompass various noisy environments ensure that the model performs well, even in the most challenging scenarios.
Adapting to Low-Resource Languages
We still face unique challenges to implementing speech recognition in low-resource languages. Sometimes, there simply isn’t enough data to have a robust training set. Finding ways to build models that learn effectively from small datasets is key to improving performance.
Inclusivity is critical, especially as more diverse languages are needed to see speech technology be inclusive and equitable.
Ensuring Data Privacy and Security
Data privacy is one of the biggest points of concern and value with AI speech recognition applications. Implementing measures like encryption and anonymization keeps user data safe while it’s being processed.
Ensuring compliance with regulations such as GDPR not only bolsters user trust but helps protect valuable data.
Reducing Latency for Real-Time Use
Of course, low latency is critical for real-time speech recognition applications. Methods such as algorithms optimization and what’s called edge computing can reduce response time to a fraction of a second.
Excessive latency drives users mad, leading to high abandonment and negative net promoter scores.
Enhancing Speech Recognition with AI
These AI advancements combine to elevate speech recognition technologies, making them more accurate, adaptable, and responsive. One of the most powerful techniques is transfer learning, which involves taking a model trained to do one thing and fine-tuning it to do something else. This reduces training time and enhances accuracy, as seen in applications like Google’s speech recognition, which leverages vast datasets from various languages. Moreover, AI speech recognition systems are increasingly benefiting from these techniques.
Self-supervised learning techniques are another major breakthrough in speech recognition ai. Specifically, here, models are trained on unlabeled data, which is more plentiful and usually more accessible. This method empowers systems to better understand underlying speech patterns, resulting in better performance across field applications.
For example, Facebook AI has been able to use self-supervised learning to improve speech recognition technology. Integrating across multiple modalities, or multimodal integration, provides even more advantages. By integrating speech recognition with visual or textual data, we can build a more dynamic, context-aware user experience.
Conversational AI agents, such as Siri and Alexa, use advanced voice recognition and contextual knowledge. AI assistants utilize this data to train their algorithms to provide more accurate responses. Lastly, a comparison between end-to-end learning and hybrid models shows clear benefits.
The end-to-end approach simplifies workflows by processing raw audio inputs, whereas hybrid models utilize conventional techniques for added versatility. Each approach has unique strengths. Specifically, while end-to-end systems might be more efficient, allowing faster speech recognition overall, hybrid systems are more of a benefit in accuracy with specialized tasks.
Ethical Considerations and Future Trends
Just as AI is revolutionizing speech recognition technology, it deepens ethical concerns. It is imperative to get ahead of bias in training datasets. When the data is biased, it often results in systems that do not perform well for those demographics, killing accuracy and user experience.
A speech recognition system built primarily on data from English speakers who are predominantly white and urban will struggle. It might have difficulty detecting accents or dialects that could be prevalent in more rural areas of a state. We can start to build more equitable datasets by blending together diverse voices and contexts, making sure that technology works better for all of us.
Accessibility is a key consideration. Speech recognition needs to be inclusive for users of all abilities, including those living with disabilities. While advances such as voice-controlled and other virtual assistants are an excellent start, there is still so much more to accomplish.
For example, adding features that help improve communication for people with speech disabilities can broaden communication and create a more inclusive experience.
Looking forward, ENGAGE AI is just getting started with our voice-enabled AI technology. We should look forward to wider adoption across industries such as healthcare, where speech recognition has the potential to reduce time spent on patient documentation.
Future innovations will need to go beyond context awareness and dynamic response and aim for human-like interactions that feel intuitive and intelligent. Ensuring that innovation is pursued responsibly must be their top priority.
To avoid unintentional or intentional harms, ethical considerations should lead the way in developing these tools. Balancing user safety and innovation while we lead on innovation, user safety is important.
Our collective commitment to this imperative will determine the future of speech recognition, creating systems that are powerful and efficient while honoring people’s rights and privacy.
Examples of AI Speech Recognition
AI speech recognition technology, particularly through Microsoft Azure Cloud, is revolutionizing how we engage with technology and each other using languages. With advancements in speech recognition algorithms, automation has significantly enhanced our ability to comprehend, translate, and replicate human speech, unlocking a plethora of new use cases in various aspects of life.
Voice Assistants and Smart Devices
Voice assistants such as Siri, Alexa, and Google Assistant give you the power to manage your world with basic commands. Request your updates directly from your assistant, or create reminders for yourself. You can tell it to play some nice relaxing jazz, just like that—all hands-free!
Smart devices—thermostats, lights, appliances, etc.—that react to voice commands make home automation easy and natural. The development trends towards ever-better voice technology are clear, with smart home ecosystems continuing to enter homes at record rates. This trend is increasing convenience and efficiency with them.
Dictation Software and Transcription Services
Using dictation software helps you be much more productive because it’s way faster to speak words into the computer and get them back as text. For busy professionals that need to write reports or emails quickly, this tool can greatly simplify the writing process.
Cost-effective transcription services play an important role in sectors such as healthcare and legal. They transcribe audio content into text for applications that serve industries like healthcare and legal. Thanks to recent technology breakthroughs, transcription tools deliver a level of accuracy and efficiency that’s been a game changer.
Today, they accurately transcribe detailed technical vocabulary with fair consistency.
Real-Time Language Translation Tools
AI speech recognition helps improve real-time translation services that further break down language barriers and create seamless cross-lingual communication. Tools including Google Translate and Microsoft Translator are making multilingual conversations more real-time and authentic than ever before.
From showcasing international business meetings to helping reunion seekers find long-lost friends and family members, these applications underscore AI’s potential to deepen human connection.
Ensuring Valuable AI-Generated Content
In an age where online accessibility is key to success, the use of AI speech recognition technology is more than just a party trick. Specifically, it seeks to ensure that AI-generated content has real added-value and usefulness for users. That starts with the requirement of delivering new ideas, thinking creatively, and being laser focused on the end user, while leveraging advanced speech recognition algorithms.
Focus on Original Insights
Content created using AI should showcase original viewpoints that distinguish it from other content. By harnessing the power of creativity, you deepen user engagement, because new unique concepts capture attention.
Techniques such as ideation workshops, challenges, or co-design with multidisciplinary teams are effective ways to foster creativity in AI use cases. For example, using more varied datasets will help you create deeper, human-centered insights that will be more likely to speak to multiple constituencies.
Prioritize User Experience
User experience is a key component in making speech recognition systems more effective. This will ultimately make the technology more accessible and enjoyable to use. Incorporating user feedback can lead to significant system improvements, making adjustments based on real-world experiences.
Clear designs provide the best experience. Clear, intuitive interfaces lead to happier users, allowing for easy on-boarding, constant use and retention.
Avoid Scaled Content Abuse
Depending entirely on scaled content generation has its own risks, including the risk of quality dilution. Avoiding a race to the bottom that values quantity over quality is critical to trustworthy AI-generated content.
Ensuring fairness and accuracy in the creation and dissemination of AI-generated content safeguards ethical practices and nurtures public trust.
Emphasize Accuracy and Reliability
In areas such as sensitive speech recognition, accuracy is non-negotiable. Systems that are dependable build trust and satisfaction among users, making them feel that every interaction will be productive and pleasing.
It’s an area where we are currently iterating on algorithms and running machine learning to maximize accuracy in AI speech technologies. These behind-the-scenes processes are key to producing reliable outcomes.
Conclusion
The application of AI in speech recognition has limitless potential. It’s revolutionizing mundane tasks helping people connect in richer, more dynamic ways. Companies benefit from improved customer experience with more granular control over voice commands, and consumers benefit from hands-free functionality. As with all technology, look for new and surprising applications that make everyday life a little easier and more efficient. Our continued emphasis on ethics helps us get these powerful tools into the world while prioritizing privacy and fairness. Keep up with these exciting new changes in AI speech technology. The more you understand how it will impact you, the better you’ll be able to grow with emerging trends and maximize the benefits in your own life or business. Learn more about this exciting space and how you can take advantage of AI in speech recognition to improve your communication.
Frequently Asked Questions
What is speech recognition?
Speech recognition AI is the underlying technology that enables computers to hear spoken words and translate them into text. This technology powers voice assistants, voice dictation, and transcription services, transforming speech into text and opening the door to innovative voice-driven experiences.
How does AI enhance speech recognition?
AI technology is revolutionizing speech recognition ai technology by employing advanced machine learning algorithms to increase accuracy and efficiency. This is what powers ai speech recognition systems to learn from millions of hours of human speech data, adapting to new accents and speech patterns.
What industries benefit from AI speech recognition?
AI speech recognition technology is having a positive impact across many sectors, such as healthcare, customer service, automotive, and entertainment, enhancing efficiencies and better serving users’ needs through advanced speech recognition capabilities.
What challenges exist in AI speech recognition?
Key challenges in AI speech recognition, such as background noise, varied accents, and understanding conversational context, significantly impact the accuracy and reliability of AI speech recognition systems in real-world applications.
What are ethical considerations in AI speech recognition?
Ethical implications such as data privacy, consent, and the potential for bias in speech recognition algorithms must be addressed. We believe that making practices fair, transparent, and accountable is key to fostering public confidence in AI applications.
Can you give examples of AI speech recognition applications?
Examples of AI speech recognition applications include virtual assistants like Siri and Alexa, as well as AI assistants in transcription services, voice-activated devices, and automated customer support systems. These speech recognition technologies enhance user experience and productivity.
How can I ensure valuable AI-generated content?
AI has the potential to generate high-quality content using speech recognition AI technology, but there are pitfalls to avoid. Prioritizing accuracy, relevance, and clarity is essential. Continuous updates and direct feedback from users can assist in streamlining the content, keeping it focused on what the intended audience truly wants and expects.
What's Your Reaction?
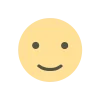
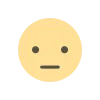
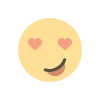
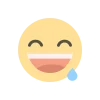
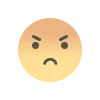
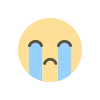
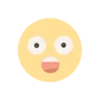