Advancements in Machine Learning and Artificial Intelligence Technologies
Explore cutting-edge advancements in machine learning and artificial intelligence, featuring unique insights and innovative technologies.
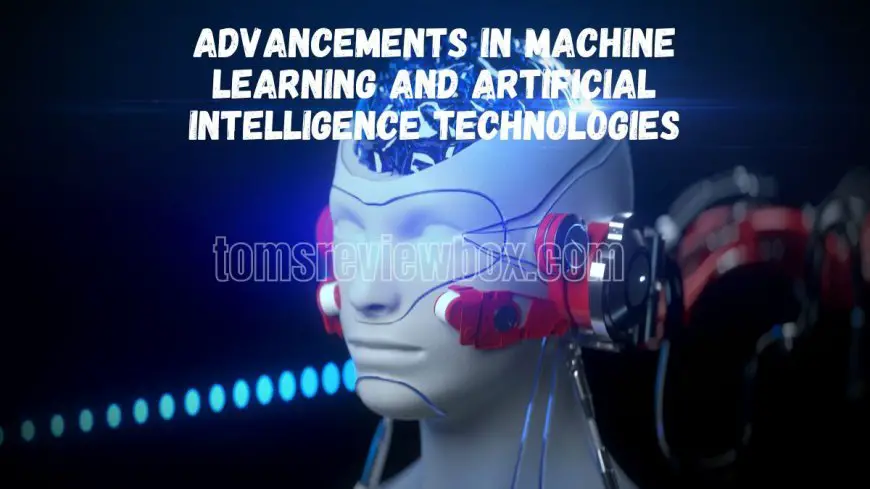
Advances in machine learning and artificial intelligence (AI) radically change the game in how we analyze data and make decisions. These technologies improve data processing speed and accuracy, allowing enterprises to surface actionable insights that fuel business innovation and growth.
In the fields of finance and healthcare, machine learning algorithms are directly predicting emerging trends. They maximize efficiency and productivity, enabling higher quality and safer outcomes for companies and their clients.
Getting up to speed on these developments is absolutely critical for those interested in being effective players in this new tech landscape. We’ll explore all the exciting new advancements in these fields.
Through practical use cases and real-world examples, we’ll show just how powerful a force they can be. With the right knowledge, you can enhance these tools to gain a competitive edge in your career and future endeavors.
Key Takeaways
- This knowledge is essential so that we can fully understand how these technologies will change the landscape of all sectors. You need to know things like accuracy and scalability to measure progress in these new fields.
- Transformer networks and generative adversarial networks are transformative technologies improving the field of natural language processing and automatic data generation. We invite you to learn more and then think about how these innovations might be adopted within your specific industry to create better outcomes.
- New advances in reinforcement learning present dynamic, smart decision-making that can be paired with automation. With the ability to use these advanced algorithms, build more efficient processes in fields such as robotics and autonomous machines.
- The importance of explainable AI goes beyond legal compliance. By implementing techniques that further model interpretability, we can safeguard trust among all stakeholders and lead the way for more responsible AI deployment.
- Ethical considerations such as bias detection and data privacy become critically important when discussing AI systems that increasingly operate in many aspects of our daily lives. We recommend that you join and lead local and national conversations about AI governance to ensure equitable technology development and use.
- Keep an eye out for state-of-the-art advancements such as quantum machine learning and zero-shot learning. These advances have extraordinary potential to transform what AI systems can do! Keeping yourself up to date will enable you to take advantage of these breakthroughs in the most useful way possible for your work.
What Defines AI and ML Advances?
Artificial Intelligence (AI) is the science of making machines simulate human intelligence, which allows them to execute functions that typically would need human cognition. Machine Learning (ML), a subset of AI, focuses on algorithms that enable systems to learn from data, enhancing performance over time. The advancements in AI and machine learning research are largely measured by the increased accuracy, efficiency, and scalability of these technologies.
Defining Artificial Intelligence (AI)
Recent innovations such as transformer networks and attention mechanisms have revolutionized the field of natural language processing (NLP). It’s these technologies that power advances in speech recognition, machine translation, and chatbots, allowing machines to engage with humans more naturally.
Graph neural networks (GNNs) have become essential tools for handling intricate relational information. They are the building blocks that allow AI systems to find patterns among disparate data sets.
Generative adversarial networks (GANs) play an important role in generating high-fidelity synthetic data. This functionality is needed to develop models by safeguarding the privacy of actual data.
Reinforcement learning algorithms continue the AI tradition of building machines that make better decisions. Reinforcement learning techniques improve decision-making through trial and error.
Defining Machine Learning (ML)
AI’s transformative potential in healthcare is particularly visible with diagnostics and personalized medicine, allowing faster and more accurate patient evaluations. In financial services, innovative uses of AI improve efficiency in the fraud detection process and enhance performance in algorithmic trading.
AI predictive maintenance, for instance, significantly boosts manufacturing productivity by an incredible 10 times. Simultaneously, AI is transforming transportation through improved logistics, especially with the advent of self-driving vehicles, creating more agile and consistent systems.
Key Metrics for Measuring Progress
Deep reinforcement learning (DRL) has been at the core of creating these smart agents, achieving superhuman performance in robotics and control applications. Advanced techniques such as sim-to-real transfer learning help close the gap between simulation and reality.
It is this innovation that renders AI applications more practical in real-world situations.
Recent Algorithmic Breakthroughs
Recent breakthroughs in artificial intelligence (AI) have had an astounding impact across sectors, dramatically increasing what’s possible in practice. Another major area of advancement is explainable AI (XAI), which plays a vital role in establishing trust and transparency in AI systems.
Techniques such as feature importance and local interpretable model-agnostic explanations (LIME) offer stakeholders transparency into the rationale of AI decision-making. This greater understanding increases their trust in the technology.
1. Transformer Networks and Attention Mechanisms
Advances in image recognition, largely powered by innovations like transformer networks and attention mechanisms, have transformed possibilities for practical applications. For instance, AI’s latest success in computer vision—specifically object detection—are contributing to advancements in industries ranging from healthcare to security.
Natural language understanding (NLU) and generation (NLG) have hit new pinnacles, with the likes of GPT-4 achieving unprecedented conversational prowess. Proven strategies to deploy these models at scale make sure devices deliver what industry needs—pronto.
2. Graph Neural Networks (GNNs)
Model compression techniques, such as quantization and pruning, are essential for improving computational efficiency while maintaining performance. GNNs have immensely profited from these techniques, enabling efficient processing of large datasets.
Edge computing and on-device AI are key to optimizing resource usage, creating a more accessible and efficient way to deploy AI.
3. Generative Adversarial Networks (GANs)
Making sure that AI systems used by our government are fair should be top priority. These bias detection and mitigation strategies are a step toward building more equitable AI.
Data privacy and security considerations are at the heart of responsible AI deployment, and governance is key to ethical AI practices.
4. Advances in Reinforcement Learning Algorithms
Clustering algorithms and self-supervised learning approaches enhance machine learning research, especially in data-poor settings.
5. AutoML: Automating Machine Learning Pipelines
With the potential for dramatically faster processing capabilities, quantum machine learning algorithms introduce new challenges and opportunities for AI research applications.
Transforming Industries with Novel AI Applications
Artificial intelligence (AI) is revolutionizing every sector known to mankind. This unprecedented integration embodies a major change in the way we think about and engage in problem solving and innovation. Integrating multiple data modalities supercharges AI systems.
This method unlocks unprecedented capabilities for AI models to leverage varied data modalities including text, images, and audio leading to more accurate, robust, and useful AI applications. The first application, for instance, in healthcare, AI can look at patient medical records in conjunction with imaging data to produce more accurate diagnoses.
A second major breakthrough is zero-shot learning. This broad generalization technique helps AI models better identify and classify unseen data, making them more adaptable. For instance, an AI trained on images of dogs and cats could still identify a rabbit based solely on its features, improving its utility in dynamic environments.
In healthcare, edge AI and TinyML simplify on-site processing, making processes faster and more efficient. Federated learning enables this decentralized model training while protecting patient privacy by keeping data on local devices. Responsible AI puts ethical considerations at the forefront, resulting in AI technologies that reflect our societal values.
In finance, AI-based algorithms perform trades after trend analysis, increasing operational efficiency while reducing potential risk. The possibility that AI will generate more jobs than it displaces, compared to jobs AI will eliminate, illustrates the double-edged nature of this technology.
AI is leading a massive transformation in sectors changing the world. It drives better outcomes in healthcare and more efficient supply chains, opening new doors with each innovation.
Enhancing Autonomous Systems with Reinforcement Learning
Reinforcement learning (RL) is an increasingly popular machine learning approach that allows machines to learn complex behaviors from their environments, significantly enhancing autonomous systems. This innovative process, known as reinforcement learning, enables agents to rapidly adjust and fine-tune their actions through feedback, showcasing the precision and adaptability of intelligent machines.
Deep Reinforcement Learning (DRL) Explained
Deep reinforcement learning (DRL) is an evolution of traditional RL, incorporating advanced deep learning techniques. Here, neural networks crunch massive amounts of data, making it possible for systems to identify intricate patterns.
For instance, in gaming, DRL has been successfully applied to train agents to play games like Go, outperforming human champions. This technique gives machines the ability to navigate complex landscapes of action and reward, resulting in more intelligent, creative, and flexible behavior.
Applications in Robotics and Control Systems
The potential applications of RL in robotics and control systems are enormous. For instance, drones use RL to autonomously navigate complex environments. Through persistent education from their setting, drones are able to enhance flight paths, steer clear of obstacles, and increase effectiveness.
In manufacturing, robotic arms leverage reinforcement learning to quickly get up to speed on a variety of tasks. This increases productivity and reduces downtime with learning in real-time.
Sim-to-Real Transfer Learning Techniques
Sim-to-real transfer learning is critical to the application of deep reinforcement learning in real-world scenarios. By using reinforcement learning to train agents in virtual environments, we are able to lower the cost and risk of training.
A real-world example is training self-driving cars in virtual traffic conditions. This ensures that they are able to learn proper driving habits before they go out on any streets. This technology largely advances the rollout of trustworthy systems in complex, ever-changing settings.
Improving Model Interpretability and Explainability
Developing model interpretability and explainability tools is quickly becoming one of the most important areas of study in artificial intelligence research. This deepened understanding will build the trust necessary to implement and use autonomous AI systems responsibly across industries and sectors.
The Importance of Explainable AI (XAI)
Explainable AI (XAI) is a term used to cover this growing need for transparency within AI systems. Whether considering a healthcare diagnosis or a loan decision, algorithms are increasingly influential in these decisions.
Stakeholders deserve clear explanations of how these conclusions are reached. For instance, a model predicting patient outcomes must provide reasons for its predictions, allowing healthcare providers to trust and validate the results. Such transparency increases accountability, and accountability is especially needed in high-stakes environments.
Techniques for Model Interpretation
There are a number of techniques available to improve model interpretation. Feature importance methods, including SHAP (SHapley Additive exPlanations), help quantify how much each feature has contributed to a model’s prediction.
For example, in a credit scoring model, SHAP can illustrate how factors like income or credit history impact the score. The second approach is Local Interpretable Model-agnostic Explanations, or LIME. This approach produces local approximations of complicated models and sheds light on individual predictions.
By leveraging these techniques, data scientists can better explain their models, allowing for easier understanding by stakeholders without the technical background.
Evaluating and Comparing XAI Methods
Evaluating XAI methods is essential to determine their effectiveness. Metrics such as fidelity are the most important type of metric because they measure how well an explanation depicts the model’s true behavior.
Invariance is another key property, measuring the stability of explanations by evaluating their consistency across similar instances. A robust evaluation framework allows organizations to select the most appropriate XAI technique for their needs, ensuring clarity and reliability in AI applications.
Scaling Computer Vision and NLP for Real-World Use
This revolution in machine learning and artificial intelligence research is changing the way we utilize computer vision. Simultaneously, it has been causing a paradigm shift in natural language processing (NLP), showcasing many AI innovations that provide real-world solutions benefiting sectors from agriculture to security through improved productivity and resource management.
Advances in Image Recognition and Object Detection
Recent advancements in computer vision technology, specifically image recognition and object detection, have allowed computers to interpret visual data with unprecedented precision. Take, for example, the impact of facial recognition technologies in building security systems to protect our assets by rapidly identifying people.
Retail companies use object detection to keep customers in stock. With this technology, they can monitor products in real time, which goes a long way in preventing stockouts and overstocking. These applications are just a few examples of how increasingly advanced machine learning models are analyzing massive quantities of visual data to drive more intelligent decision-making.
Natural Language Understanding (NLU) and Generation (NLG)
Natural language understanding and generation is at the center of human-computer interaction. Using advanced algorithms, these systems are able to understand context, sentiment, and intent behind a user’s query.
Chatbots in customer service are a great example of this—offering instant responses and solving problems with no employee involvement. Content generation tools, like intellifusion, generate relevant articles or reports based on user inputs, reducing workflow bottlenecks and increasing productivity.
Deploying Vision and Language Models at Scale
Scaling these models for real-world use poses challenges such as computational demands and data privacy concerns. Through cloud compute power, companies are using these technologies to democratize and disperse processing power, putting advanced analytics in the hands of more users than ever.
Healthcare providers leverage such models to analyze complex patient records to suggest courses of treatment and predict outcomes. This improves clinicians’ treatment plans, all while maintaining patient privacy.
Optimizing Models for Resource Efficiency
With the evolution of machine learning and artificial intelligence, optimizing these models to be resource-efficient has become a critical part of the process in artificial intelligence research. This combination of high-performance priority and resource efficiency easily translates into lowered cost of operation, which is essential for data scientists and AI engineers. You can achieve this through methods like model compression, quantization, and utilizing edge computing.
Model Compression Techniques
Model compression is a key technique to make machine learning models smaller and more efficient while maintaining the same level of accuracy. Techniques such as weight sharing and knowledge distillation contribute to finding smaller models.
Even still, these models retain all the core functionality and features of their full-size siblings. For example, a deep learning model for image recognition can be compressed by as much as 91 times. Despite this, it packs a ton of accuracy under the hood.
This greatly reduces the practical feasibility of deploying such a model in resource-constrained environments.
Quantization and Pruning Methods
Quantization and pruning are two robust techniques to achieve efficiency. Quantization reduces the number of bits required to represent weights. Consequently, this improves the computation time and memory consumption.
Quantization such as converting 32-bit floating point numbers to 8-bit integers can greatly increase the inference speed of a model. While distillation focuses on training a smaller model, pruning is the act of removing redundant, unimportant weights from your model.
This shortens the model and makes it faster to process as well. For instance, a model initially containing millions of parameters can be pruned down to hundreds of thousands, achieving a lighter and faster version.
Edge Computing and On-Device AI
Edge computing is really catching on! This makes it possible to deploy AI models to run directly on devices, enabling real-time processing on-the-fly and greatly minimizes latency in decision making.
Now, devices like smartphones or IoT sensors are capable of running sophisticated AI models on-device. This functionality gives users real-time visibility while minimizing their dependence on internet connections.
For example, a smart camera can analyze video feeds directly, leading to quicker decision-making without sending data to a central server.
Addressing Ethical and Regulatory Challenges
As machine learning and artificial intelligence emerge, ethical and regulatory challenges, particularly in AI ethics, rear their ugly heads. Continuing development of these technologies is vital to address equity concerns and ensure responsible deployment, maximizing the benefits of AI technologies.
Bias Detection and Mitigation in AI Systems
Bias introduced in these AI systems can result in discriminatory outcomes, affecting decisions made during hiring, lending, and law enforcement, among other examples. A clinical AI model trained on historical data will learn these societal biases. As a result, it risks reinforcing those biases.
Rectifying and preventing these biases requires iterative testing and varied datasets. Tools like adversarial debiasing and fairness constraints can go a long way toward producing more fair algorithms. By making an intentional effort to prioritize fairness, we can begin to build greater trust in these AI applications.
Data Privacy and Security Considerations
Data privacy is an essential aspect of AI considering the large amounts of sensitive, personal information that is used to train models. Organizations should ensure data protection approaches such as encryption or anonymization are part of their user data protection practices.
Compliance with regulations like the General Data Protection Regulation (GDPR) ensures that individuals' rights are respected while harnessing data for innovative applications. Routine audits improve compliance and help detect and mitigate potential vulnerabilities, making data security a constant consideration rather than an afterthought.
The Role of AI Governance and Regulation
With AI’s rapid evolution in mind, we must continue to build robust governance frameworks. Providing broad but specific guidelines and standards helps set expectations to mitigate risks when developing and using AI technologies.
Collaboration among all stakeholders—including governments, industry leaders, and researchers—will be key for developing effective regulatory frameworks. These frameworks should encourage innovation while upholding strong accountability and transparency standards in AI systems.
By committing to a culture of responsible AI development and application, we can make meaningful strides through this dynamic field.
Unsupervised and Semi-Supervised Learning Improvements
Recent advancements in unsupervised and semi-supervised learning have significantly enhanced our ability to analyze complex datasets in machine learning research without extensive labeled data. These advanced techniques allow us to discover unexpected patterns and insights, contributing to the ongoing evolution of artificial intelligence research.
Clustering Algorithms and Dimensionality Reduction
Clustering algorithms, like K-means clustering and hierarchical clustering, play an important role in unsupervised or semi-supervised learning. For example, in customer segmentation, these algorithms allow companies to segment customers and target their marketing efforts accordingly.
Techniques such as t-SNE or PCA (dimensionality reduction techniques) reduce the data from high-dimensionality to only a few dimensions, allowing us to visualize it. Through aggressive noise reduction, synthetic feature generation, and heuristic feature creation, we will increase model performance and interpretability.
Self-Supervised Learning Approaches
Self-supervised learning has become increasingly popular, allowing models to learn from unlabeled data through the process of creating their own labels. This method has proven effective in areas such as NLP and computer vision.
For instance, models such as GPT-3 leverage massive datasets of text gathered from the internet to understand context and semantics without the need for labeled data. This has the effect of minimizing the need for expensive, specialized labeled datasets, which significantly helps with the training process and widens the range of applications.
Applications in Data-Scarce Environments
These learning strategies are especially powerful in data-scarce environments, like medical imaging, where annotated data may be difficult to come by. They let us take advantage of limited amounts of labeled data in addition to massive unlabeled datasets.
For instance, semi-supervised learning can improve diagnostic accuracy in rare diseases by training models with limited patient data and vast unlabeled scans.
Quantum Computing's Potential Impact on AI
Quantum computing has incredible potential to transform artificial intelligence research, which relies heavily on data processing speed and the complexity of calculations. Combining quantum mechanics with machine learning skills can ignite significant advancements in this field. This innovative solution will change the way we look at data and uncover insights.
Quantum Machine Learning Algorithms
Quantum machine learning algorithms use quantum bits, or qubits, to undertake calculations that would take classical computers inordinate amounts of time to complete. For example, the Harrow-Hassidim-Lloyd algorithm demonstrates that quantum systems can solve linear systems exponentially faster than classical counterparts.
This potential would enable improved predictive analysis and optimization across multiple domains, such as finance and healthcare. By leveraging these algorithms, we’ll make better predictions and make better decisions as a result.
Challenges and Opportunities in Quantum AI
Despite the exciting potential, a lot of challenges still remain. Quantum hardware is so nascent that it’s hard to imagine a widespread application. High error rates in qubit operations can result in erroneous outputs, representing an enormous challenge.
Yet, all these challenges create moments of opportunity to innovate. Creating error-tolerant quantum systems and building out the best quantum algorithms will open doors to many practical applications. Partnerships between industry leaders and research centers will speed up progress, producing an environment ripe for revolutionary developments in quantum AI.
The Future of Quantum-Accelerated AI
Looking to the future, there are such profound solutions quantum-accelerated AI can unlock that we can’t even yet conceive of them. Perhaps the next great breakthroughs will be in drug discovery, optimizing logistics, or improving cybersecurity.
Together, quantum computing and AI will continue to transform industries, enhancing productivity and impact. With further research, the real-world applications will be made clearer and more impactful.
Multi-Modal and Zero-Shot Learning
The mammoth fields of machine learning and artificial intelligence research progress so swiftly, with recent advancements in multi-modal learning and zero-shot learning accelerating this change. These innovative solutions enable intelligent machines to analyze, understand, and act upon various data modalities, unlocking exceptional capabilities.
Combining Different Data Modalities
Multi-modal learning combines different modes of data, including text, images, and audio. This method allows for fewer mistakes by AI systems, leading to better decision-making and more reliable AI systems.
A model taught using both text and images develops a more nuanced comprehension of a product. It accomplishes this by performing joint review and image-based analysis. This results in far more precise predictions and deeper insights.
AI-powered, voice-first assistants directly respond to complex, spoken commands and requests. They process visual cues from the context provided by their environment.
Zero-Shot Learning Techniques
Zero-shot learning enables models to identify and label information from completely new categories that they’ve never encountered. This becomes especially powerful in settings where you have limited labeled data.
For example, an AI can learn to recognize a zebra by understanding what makes it a zebra. It doesn’t require any previous samples to fit the rule! This ability dramatically reduces the requirement for massive training datasets.
The result is rapidly and easily deploying AI in all kinds of applications.
Enhancing Adaptability in AI Systems
When paired together, multi-modal and zero-shot learning greatly improves the adaptability of AI systems. These systems can learn from new information and adjust their performance accordingly, which is crucial in dynamic environments like finance or healthcare.
Through the use of these techniques, organizations can future-proof their AI and maintain systems that are effective and relevant even as the world around them shifts.
Trends in AI and Machine Learning
The trends in artificial intelligence (AI) and machine learning (ML) outlined below are already having a major impact and are just the beginning. These major trends are creating an environment that is making it easier, cheaper and faster for these technologies to integrate into all sectors of the economy.
Only by understanding these trends can you hope to successfully capitalize on their tremendous promise.
Edge AI and TinyML
Edge AI is a term for processing data directly on devices, as opposed to mostly on centralized cloud infrastructures. This approach uses less latency and bandwidth and provides users with faster and more responsive applications.
For example, with smart cameras, facial recognition can be processed on the camera itself in real-time, allowing for immediate response without waiting on the cloud. TinyML, a subfield of Edge AI, is concerned with executing machine learning algorithms on such small, low-power devices.
Now picture smart sensors in your home that are capable of analyzing environmental data while maintaining a local database that doesn’t require continuous internet connection. This more widespread connectivity and increased processing power opens the door to big-league capabilities in everything from wearables to industrial IoT devices.
Federated Learning
With federated learning, many different devices may collectively do the work of training powerful machine learning models without ever bringing data to a central point. Consider your smartphone, which applies AI to learn your habits – all while preserving your privacy.
The model gets stronger without having to transmit your sensitive data to a central server. This approach enhances privacy by reducing data transfer costs. In a world increasingly concerned with protecting the data we all share, these benefits are more important than ever.
Responsible AI and Ethical Considerations
As AI technologies continue to expand, ensuring responsible use grows increasingly urgent. Ethical considerations include making sure algorithms are unbiased and interpretable.
Unintended negative consequences include favoring unfair hiring opportunities due to algorithmic bias. By adopting principles for ethical use of AI, including an emphasis on equity and accountability, developers can make sure systems they deploy foster equity and accountability.
Future Use of Artificial Intelligence
Artificial intelligence (AI) has the potential to dramatically change public and private sectors alike—improving efficiency, deriving new insights, and developing novel solutions. Engaging with AI research and understanding its future applications is critical for your own learning and ultimately, your career.
AI-Driven Scientific Discovery
AI is revolutionizing scientific research, from speeding up data analysis to building models of complex systems. As an example, AI’s role in drug discovery has surged, where AI algorithms can process extensive datasets to recommend promising compounds at an accelerated pace compared to conventional approaches.
Researchers at institutions like MIT use machine learning to predict molecular interactions, significantly shortening the time required for developing new treatments.
Personalized Education with AI
In education, AI personalizes learning experiences, adapting to each student’s unique strengths and challenges. Platforms like Coursera and Khan Academy use AI to track student progress and modify courses in response.
This tailored experience not only allows students to better understand difficult concepts, but nurtures a sense of curiosity and interest in the subject matter. Educators see the benefits too, receiving reports on what students are doing well on and what needs more focus.
AI for Sustainability and Environmental Protection
AI can help make sustainability initiatives greener and more effective by identifying opportunities to conserve resources and minimize waste. Smart grids, AI-driven, improve energy efficiency by forecasting energy demand and optimizing supply to meet it.
Google’s initiatives probably use AI to make data centers 300 times more energy efficient. This has proven to be a powerful and cost-effective approach to drastically reduce carbon footprints.
In addition, AI has the potential to monitor ecosystems, allowing conservationists to keep tabs on endangered species and determine overall habitat health.
Conclusion
Looking forward, electrifying and then plugging into the advances in machine learning and artificial intelligence would create doors to opportunities we can only imagine. You can witness the momentous tide of algorithmic and application breakthroughs that are disrupting virtually every industry you observe, creating more fluid, seamless, smarter and efficient processes. AI deepens our understanding and democratizes technology. Cutting-edge developments in these areas, especially for autonomous systems and their interpretability, expand the frontiers of possibility. Resource efficiency and ethical considerations will continue to empower and inform all our paths ahead. To be clear, the picture is not all dark. The future is really bright with quantum computing and new learning approaches. All of these changes are felt in our everyday lives and professions. You’ll be better positioned to stay ahead if you familiarize yourself with these trends and find ways to incorporate them into your work. Explore the exciting new world of AI and machine learning, and follow your interests where they take you.
Frequently Asked Questions
What are the main advancements in AI and ML?
Recent breakthroughs in artificial intelligence research have been focused on developing state-of-the-art algorithms, enhancing feature selection, and improving resource efficiency. These advances make technology more equitable, explainable, efficient, and scalable — unlocking their power for organizations in every sector, from healthcare to financial services.
How are algorithms advancing in AI?
Recent breakthroughs in algorithms, such as transformers and generative models, have significantly improved performance in tasks like image recognition and natural language processing, leading to more accurate and efficient artificial intelligence research systems.
What industries are being transformed by AI?
AI is transforming industries from healthcare to finance, transportation to retail, with significant advancements in predictive analytics and autonomous systems, enhancing workflows and decision-making in various fields.
What is reinforcement learning?
Reinforcement learning, a crucial aspect of machine learning research, enables intelligent agents to learn through trial-and-error with their environment. This optimization of decision-making is vital for autonomous systems, particularly in applications like self-driving cars and robotics, showcasing many AI innovations.
Why is model interpretability important?
By making artificial intelligence research more understandable and transparent, model interpretability helps build trust and accountability. Both are key ingredients to responsibly deploying such powerful technology, especially in sensitive fields such as healthcare, where understanding why a decision was made can significantly impact patient outcomes.
How does quantum computing impact AI?
Quantum computing could supercharge AI capabilities, rapidly increasing the speed of more complex calculations. This has the potential to drive significant advancements in machine learning research and understanding data at scale.
What is zero-shot learning?
Zero-shot learning enables artificial intelligence models to identify objects or concepts they were not specifically trained for, enhancing the machine learning skills necessary for adapting to emerging challenges efficiently, thus reducing the need for major retraining.
What's Your Reaction?
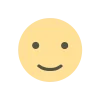
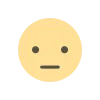
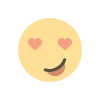
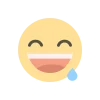
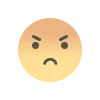
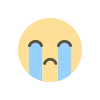
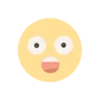