Top 10 Applications of AI in Natural Language Processing
Discover the top 10 innovative applications of AI in natural language processing that transform communication and data analysis.
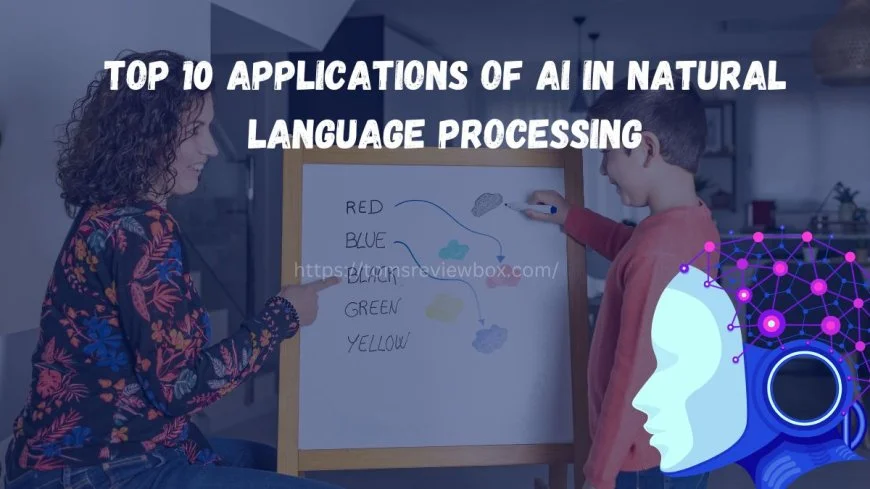
Key Takeaways
- Natural Language Processing (NLP) allows computers to understand and interpret human language, bridging communication gaps. Learning the implications of it can improve the relation between humans and machines in the daily life activities.
- AI is at the heart of NLP, bringing new levels of understanding and generative language capabilities to our applications. As your skill level increases and technology progresses, you can use AI to develop more complex applications that react intelligently to user input.
- Get acquainted with fundamental concepts in NLP like tokenization and semantic analysis. This understanding will put you in a much stronger position to understand how NLP systems work and the difference they can – and can’t – make across industries.
- AI technology has practical applications in natural language processing widely across every sector from healthcare to finance. As you navigate these domains, you’ll identify ways to leverage NLP processes to improve customer experience, drive productivity, and create more efficient, tech-enabled operations.
- Understand the limitations of NLP, including data bias and computational demands. Recognizing these problems is a key step toward designing responsible, fair, and secure NLP systems.
- Watch for new trends such as explainable AI and multimodal NLP. Keeping abreast of more recent advancements can help spark creative new ways to use language technology to tackle the world’s challenges.
The transformative impact of AI on natural language processing (NLP) is changing the game for all human-computer interactions. AI deepens machines’ understanding and generation of human language, furthering their ability to engage in authentic conversations.
From virtual assistants simplifying our daily routines to chatbots enhancing customer service, the effects are profound. AI powers sentiment analysis for social media monitoring and even real-time translation, helping people communicate better across cultural and linguistic barriers.
As a lover of the finer details of language, I find it captivating. AI helps us distill complex interactions into simple exchanges and intuitive experiences.
What is Natural Language Processing?
Natural Language Processing, or NLP, is one of the most interesting areas of artificial intelligence. That’s because it underpins every human-computer interaction we know today. This technology is a gap between languages, cultures, and communication needs.
It improves interactions between humans and machines, making things more seamless and easier to use. Through the study of language structures, NLP improves the way humans interact with computers, powering tools from simple chatbots to complex document search engines.
Define Natural Language Processing
At its most basic, “natural language” is the organic, informal language that we all use. NLP is a branch of AI that aims to comprehend and create this human language.
Applications such as automated customer service chatbots can be especially important, as they provide dynamic, real-time responses to user queries. They all show how NLP can effectively automate tedious tasks, like processing legal documents, greatly reducing human error.
For many consumers, natural language processing is a technology they use every day, oftentimes without even knowing it.
NLP's Relationship with Artificial Intelligence
NLP has become a critical part of AI technology, enabling machines to learn, understand and respond to human language in a valuable way. The combination of NLP with advancements in machine learning takes language understanding to new levels, allowing machines to understand context, things like sarcasm, or metaphors.
With AI advancements come NLP technologies, constantly enhancing their abilities.
Key Components of NLP
NLP encompasses many important aspects, including tokenization, parsing, and semantic analysis, where algorithms and models interpret and generate natural language using sophisticated language rules and common NLP tasks.
Stages of Natural Language Processing
The stages of NLP include:
- Data Collection
- Text Preprocessing
- Feature Extraction
- Model Training
Together, each stage serves to enhance the power and precision of NLP applications, creating an exciting and dynamic frontier in this quickly evolving space.
AI's Role in Transforming NLP
AI has had a transformative role in NLP’s evolution. AI enhances NLP applications and services through powerful algorithms and machine learning methods. This augmentation is a game changer for the deep learning NLP space.
For example, AI can rapidly compare millions of instances of language, discovering patterns of use in a way similar to the way a child learns. This kind of intuitive understanding opens up new possibilities for communicating more effectively whether you’re on Facebook or on Skype.
1. Enhance Language Understanding with AI
AI models do a far better job of understanding context and semantics. Deep learning techniques allow systems to understand subtleties in human language.
The quality of training data becomes extremely important, since high-quality data over-index on quality understanding. That’s one of the reasons tools such as Grammarly improve quality by factoring in intent, helping users write with greater clarity and precision.
2. Improve Language Generation with AI
AI-driven models are powerful tools when it comes to producing coherent, contextually relevant text-at scale. This talent is prominently on display in chatbots and virtual assistants such as Amazon’s Alexa and Apple’s Siri, which offer human-like, contextually appropriate responses.
Recent advancements in Natural Language Generation (NLG) technologies allow for richer, more conversational experiences.
3. Overcome Ambiguity with AI Models
AI helps with tasks like efficiently disambiguating words and phrases that have multiple meanings. With context a key component, models are able to disambiguate meaning with precision.
Through machine learning, these models learn to understand these linguistic subtleties, creating more effective user experiences.
4. Contextual Understanding in Multilingual NLP
Secondly, AI enables multilingual NLP by allowing systems to understand context over many different languages. Further challenges come from the necessity to maintain contextual integrity during translations, revealing that applications must be culturally conscious.
This is necessary to develop truly effective multilingual tools that are culturally appropriate and relevant to diverse audiences.
Explore NLP Applications Across Industries
On a broader scale, NLP has been increasingly adopted across industries, streamlining processes and unlocking innovative functionalities. Its influence is enormous—with wide-ranging applications in healthcare, finance, customer service, education, and marketing.
From health care to transportation to media, these sectors are reaping the rewards from NLP’s power to process human language and uncover important information.
NLP in Healthcare
In the realm of healthcare, NLP assists in evaluating patient records, extracting critical information to enhance the accuracy of diagnosis and treatment strategies. For example, it can process terabytes of clinical notes and pull out essential information, allowing focus on the most critical patients and improving patient care.
NLP has transformed the landscape of medical transcription and clinical documentation. It improves speed and precision, both critical in the high-stakes world of medical environments.
NLP in Finance
In finance, NLP is instrumental in analyzing financial reports and gauging market sentiment, influencing trading decisions. It plays a role in fraud detection and risk assessment, identifying patterns that might go unnoticed.
NLP automates compliance processes, ensuring businesses adhere to regulations without manual oversight.
NLP in Customer Service
NLP fuels chatbots and virtual assistants, making customer service more efficient and accessible. These automated chatbots streamline customer interactions, improving efficiency while offering targeted, personalized recommendations that customers value and engage with.
Sentiment analysis at 90% accuracy saves businesses time and resources by getting a pulse on customer thoughts and feelings to make more informed service adjustments.
NLP in Marketing and Sales
In the realm of marketing, NLP understands consumer trends and preferences, helping target advertisements and personalize content. This powerful technology helps businesses make the most of their marketing dollars with intelligent, data-driven strategies.
These strategies help brands reach their target audiences in more meaningful ways.
NLP in Education
In education, NLP tools are improving the learning experience by analyzing student performance and providing tailored recommendations. These powerful NLP applications assist teachers in grading essays and delivering personalized feedback, significantly enhancing student success.
Practical Use Cases of AI-Driven NLP
AI-driven NLP has revolutionized almost every industry, creating seamless and efficient human-computer interactions through powerful NLP tools. From customer service to marketing, the possibilities of NLP improve customer interactions and enhance operations significantly.
AI Chatbots and Virtual Assistants
AI chatbots employ NLP technology to interact in a human-like conversational style. They answer questions, share insights, and suggest actions. In customer support, chatbots can manage thousands of inquiries at once, providing instant responses and leading to higher user satisfaction.
Thanks to recent breakthroughs in AI, these virtual assistants can comprehend context like never before, creating interactions that are much more natural.
Sentiment Analysis and Opinion Mining
NLP serves as a barometer of public sentiment by conducting a social media analysis. For brands, tracking the public conversation around their release is crucial to prioritize consumer sentiment and adapt their marketing efforts accordingly.
By leveraging sentiment analysis, businesses can be more proactive in their responses to customer feedback, leading to improved brand loyalty and customer engagement.
Language Translation Services
AI-powered NLP allows for communication in any language seamlessly, opening the door to a global talent pool. Tools such as Google Translate have made great strides with the help of machine learning.
Even with these developments, it’s still a challenge to keep multi-turn context and idiomatic expression intact while translating.
Text Summarization and Information Extraction
NLP techniques can take long reports and summarize them into actionable bullets. This is especially important for the analytics and reporting functions that are necessary to save time and improve data-informed decision making.
By automating summarization processes, businesses can spend their efforts on deriving actionable insights instead of sifting through data.
Voice Recognition and Speech-to-Text
NLP fuels modern voice recognition systems that recognize what you say and turn speech into text. Practical use cases for this technology have obvious, hugely successful applications in customer service, transcription, and accessibility tools.
Ongoing improvements in NLP are increasing the precision of these systems, helping to render them more trustworthy.
Emerging AI Techniques in NLP
The world of natural language processing (NLP) is moving at lightning speed thanks to emerging AI techniques, particularly in speech recognition and smart assistants. These innovations are key to pushing the frontier of how well machines read and write text like people do.
Transformer Networks
Transformer networks have transformed modern NLP applications. They enable neural models to better model sequential information like sentences, learning complex contextual information and better modeling relationships between words.
As an example, with translating different languages, transformers translate sentence by sentence instead of word for word, producing a more precise translation. The architecture increases performance by allowing for matrix operations to be processed in parallel, drastically increasing efficiency and speed.
BERT and its Variants
The BERT transformer model changed the game for contextual understanding in NLP. By considering the surrounding words of a target word, BERT grasps nuanced meanings, making it invaluable for tasks like sentiment analysis.
Variants such as RoBERTa and DistilBERT serve specialized applications, reducing model size while maintaining peak performance. BERT’s innovations have broken new ground across the board of natural language understanding tasks.
GPT Models and Large Language Models
One of the areas where GPT models really shine is content creation. They’ve made their way into the creative writing process, too—from drafting news articles to creating poetry.
These massive LLMs supercharge conversational AI. They enhance communication experiences, enabling a more human-like exchange and setting the stage for truly human-like conversational agents.
Reinforcement Learning for NLP
Reinforcement learning is an effective approach to train NLP models, improving their decision-making skills. This method enables models to iteratively learn through user interactions, thus facilitating ever higher performance.
The potential for developing intelligent conversational agents through reinforcement learning is immense, creating systems that adapt and respond more effectively.
Challenges and Limitations of AI in NLP
Integrating AI into natural language processing (NLP) and its common NLP tasks, such as speech recognition and semantic search, arguably poses the greatest challenge. We need to tackle issues like bias and accountability head-on to unlock AI’s full promise, ensuring responsible development in the field.
Data Dependency and Bias
Data is one of the most important factors underlying the success of NLP models. Large, high-quality, diverse datasets are key to making sure models are able to understand the nuances of language effectively. When datasets are prejudiced, the results can end up mirroring those biases, producing slanted interpretations or replies.
An NLP model trained exclusively on text from a single demographic would struggle. It frequently fails on counting processing accurately language from outside of our own culture. This highlights the importance of creating systems with varied data to create equitable systems. It further seeks to highlight the ongoing work needed to redress inherent biases in language processing technologies.
Computational Complexity
In addition, training and deploying NLP models are incredibly computationally expensive. As datasets increase in size so too does the need for processing power. This can limit scalability and accessibility, a critical factor for many smaller organizations.
Finding the best algorithms becomes vital given the scale of these complexities. Optimizing algorithms is necessary to cut through the noise, deliver relevant NLP solutions effectively, and avoid burdening resources.
Ethical Considerations and Privacy
Using NLP—especially in the analysis of large amounts of data—opens up immense ethical responsibilities. Dealing with sensitive language data means considering privacy from the ground up, keeping user information safe and private.
Responsible AI practices are essential to prevent harmful misuse of advanced language processing technologies and to earn user trust.
Handling Nuance and Sarcasm
Understanding subtle forms of expression and irony creates distinct hurdles for NLP. Without the subtleties of context that make human communication so rich and nuanced, AI can easily falter.
To create experiences that feel more natural and contextual, recent breakthroughs focus on broadening NLP’s understanding of these nuances. This approach aims to increase overall understanding and user experience.
Measuring the Impact of AI-Based NLP
To truly grasp the magnitude of AI-based NLP’s impact, particularly in common NLP applications like speech recognition and , you have to dig deep into a few critical stats. These are battle-tested metrics that can give you a reality check on what’s working with your NLP projects, what isn’t, and what can be improved.
Key Performance Indicators (KPIs)
When assessing NLP initiatives, focus on these essential KPIs:
- Accuracy: Measures how correctly the system interprets and processes language.
- Captures user feedback on their experience with the NLP application.
- Evaluates how quickly the system responds to user inputs.
- Cost Efficiency: Analyzes the cost savings achieved through automation.
These KPIs help shape your strategy, pointing you to areas where you need to double down and improve performance across the board.
ROI of NLP Implementations
Measuring ROI requires you to be able to calculate the financial benefits of using NLP and compare that to what it costs you. Factors affecting this balance are the cost of implementation, cost savings from operational efficiencies, and overall user satisfaction.
This was certainly the case for one company that deployed AI-based NLP in customer service, resulting in an impressive 40% response time decrease. This improvement dramatically increased customer retention and revenue.
User Engagement Metrics
Engagement metrics, such as interaction rates, session durations, and conversational drop-off points, can help you understand how users are engaging with your NLP systems. Tracking these can reveal specific areas where things can be improved.
User feedback is immensely important. It has allowed us to continually refine functionalities and customize the system to best suit user needs.
Accuracy and Efficiency Gains
AI-powered NLP transforms tasks involving language on any scale with remarkable precision and clarity, creating more effective connections and interactions.
Better automation from AI-powered algorithms increases efficiency while having a larger positive influence on business performance.
Future Trends and Research Directions
As we delve into what’s next for Natural Language Processing (NLP), a few key trends are influencing and shifting the future landscape. These trends, while challenging the boundaries of what language technologies can achieve, ensure a much broader, inclusive landscape.
Ongoing research is critical to push these technologies forward, making sure they are flexible enough to meet the demands of our dynamic communication landscape.
Low-Resource Language NLP
Creating NLP solutions for such low-resource languages is a formidable challenge. With little data available to train models on, many languages are missed out. Subsidiarity inclusivity is vital in language technology.
Without it, we actively perpetuate the marginalization of speakers of these languages. Initiatives like the African Language Technology Initiative are working to improve NLP capabilities for underrepresented languages, ensuring that diverse voices are heard and understood.
Explainable AI (XAI) in NLP
Explainable AI, or XAI, is becoming an important part of the machine learning ecosystem. Engaging and transparent processes create a sense of ownership and trust between users and the engagement process.
When users understand how a model has come to its conclusions, they are more likely to accept AI-driven language technologies. Further development and ongoing research into XAI can lead to increased user trust in these systems, setting the stage for greater adoption.
Neuro-Symbolic NLP
Neuro-symbolic approaches, which integrate neural networks with symbolic reasoning, are cultivating new depths to language processing capabilities. This powerful combination enables deeper understanding and reasoning to tackle complex language tasks more comprehensively.
Utilizing the synergy of natural language and knowledge graphs, we empower next generation NLP systems to become more flexible, controllable and smarter.
Multimodal NLP
Multimodal NLP, which combines text, speech, and visual data, offers a richer and more engaging user experience. This method opens the door for far more nuanced readings of context and intent, adding depth to communication across mediums.
The possibilities of these multimodal systems are truly exciting, with the potential to dramatically improve how we work, learn and communicate.
Conclusion
AI is changing the way we interact with language. It fuels creativity, promotes discovery, and leads to innovation in all disciplines. From chatbots that help customers to tools that process data, AI-powered NLP offers new levels of speed and accuracy. You’re witnessing it across the board, from healthcare to finance to marketing, streamlining processes and allowing for quicker, smarter execution. The outlook is even more encouraging, with new and improved techniques just over the horizon. These innovations not only hold the key to dramatic changes in the way we harness language. As these trends continue to re-shape the market, understanding them is key to staying one step ahead. So take a plunge into this magical world and welcome the disruption. Learn how AI can open new possibilities in your work and your everyday life. Follow your curiosity and explore the magic of words today in ways you’ve never imagined.
Frequently Asked Questions
What is Natural Language Processing (NLP)?
Natural Language Processing (NLP) is one of the most exciting areas of artificial intelligence, combining computational linguistics and machine learning techniques. It enhances user interaction by enabling machines to understand human speech and respond in a conversational manner, making it essential for smart assistants and text analytics.
How does AI transform NLP?
AI powers NLP by opening up new avenues for machine learning algorithms that learn from data, enhancing natural language capabilities. As a result, these technologies are enabling better language comprehension and automated reply generation, creating increasingly seamless user experiences with sophisticated language understanding.
What are common applications of NLP in industries?
NLP is widely used in sectors like healthcare for patient interaction, finance for sentiment analysis in trading, and customer service for chatbots, leveraging powerful NLP tools. Not only do these applications save valuable time and resources, but they create holistic, user-friendly processes.
What are some practical use cases of AI-driven NLP?
Virtual assistants such as Siri and Alexa, along with powerful NLP tools like automatic translation services and content generation tools, are prime examples of how speech recognition can save time and boost productivity.
What emerging techniques are influencing NLP?
Cutting edge technologies such as transformer models, including BERT and GPT, are revolutionizing the world of NLP by enhancing contextual understanding and enabling powerful NLP applications that generate highly human-like text, making interactions with AI more natural and intuitive.
What challenges does AI face in NLP?
AI in NLP must navigate challenges like language ambiguity, cultural context, and bias in training data to ensure accurate speech recognition and equitable natural language capabilities in various applications.
What future trends can we expect in NLP?
Future trends in NLP research might involve even more personalization, real-time translation through smart assistants, and improved emotion detection, making AI central to how we communicate with voice assistants.
What's Your Reaction?
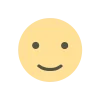
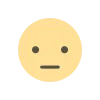
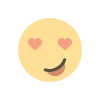
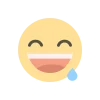
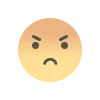
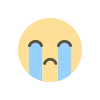
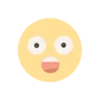