Artificial Intelligence Applications in Optimizing Power System Operations
Discover innovative applications of AI in power systems, enhancing efficiency and reliability for a sustainable future.
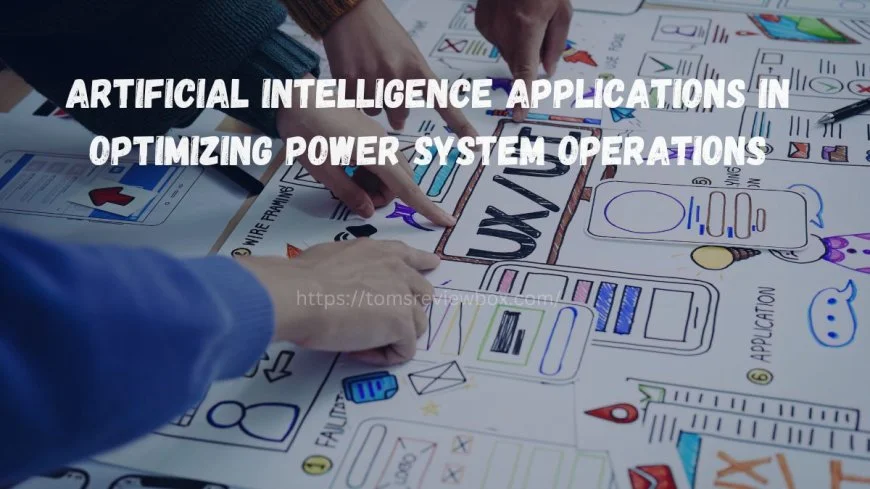
Key Takeaways
- Deploy AI algorithms to monitor grid conditions in real-time, predicting and preventing outages, improving overall grid reliability and reducing downtime.
- Use AI to dynamically manage and optimize grid resources and energy distribution in real-time. This bottom up approach scales energy efficiency and reduces emissions in a smarter way.
- Implement AI-driven predictive maintenance strategies to forecast potential equipment failures. This approach identifies actions that will extend the lifespan of our power assets and saves money.
- Leverage AI to identify anomalies in energy consumption. This method allows for outlier detection at an early stage, allowing detection of unusual trends that are indicative of upcoming problems.
- Discover AI-driven innovations to enhance efficiency and effectiveness of power system operations, encouraging new partnerships between technology developers and energy practitioners.
- Be aware of other trends, such as edge computing and digital twins. Whether or not these innovations make AI much more capable of power system management remains to be seen.
The use of AI in power system operation increases both efficiency and reliability. With the help of AI, utility companies can better forecast demand, allocate resources and improve grid operations.
With these advanced tools such as machine learning and data analytics, AI plays a pivotal role in pattern recognition and defect prediction before they turn into bigger problems. That translates into fewer, shorter outages and a more reliable energy supply for consumers.
AI facilitates a deeper integration of renewable energy sources, ensuring power systems are more sustainable. Understanding how AI works in these systems can open doors to innovations that improve our daily lives and the environment.
AI Enhances Power Systems
AI-assisted procedures, particularly those utilizing generative AI, represent a major leap forward for electric power systems, addressing the grid’s growing need for efficiency and reliability.
1. Improve Grid Reliability with AI
AI technologies create an overarching view of grid conditions, predicting potential outages and failure points with near-impeccable precision. By applying machine learning models on the historical data available, the decision-making process for managing the grid is enhanced.
AI-driven simulations can better test how the grid would respond to several different scenarios, improving reliability across the board. With automated response systems, grid failures are resolved rapidly, reducing downtime and maintaining a consistent power supply.
2. Optimize Energy Efficiency with AI
AI looks at patterns in energy consumption, recommending efficiency improvements based on individual needs. These systems in turn control and optimize energy distribution dynamically based on real-time demand, ensuring that resources are used most effectively.
By predicting energy loads with precision, AI aids in keeping energy generation in balance, minimizing waste and the need for excess production.
3. AI for Predictive Maintenance
By employing AI algorithms, equipment health data can be analyzed in order to proactively predict maintenance needs before failures happen. Condition-based monitoring saves money and increases the lifespan and reliability of power assets.
By scheduling costly maintenance during off-peak hours, predictive analytics help eliminate downtime, which means operations continue without interruption.
4. Enhance Anomaly Detection with AI
AI-powered models, particularly those utilizing deep learning techniques, can significantly enhance fault detection in electricity networks by identifying unusual patterns in energy consumption. This capability allows for real-time reporting and swift responses to irregularities, ensuring a more reliable power system protection.
Machine Learning Applications
Machine learning, particularly through generative AI support systems, has the potential to revolutionize every aspect of how we operate electric power systems, increasing system efficiency and supporting better decisions. With applications ranging from predictive maintenance to cybersecurity, it plays a crucial role in predictive analytics and grid optimization.
Supervised Learning for Power Systems
At the core of predicting equipment failures through analysis of historical data is supervised learning. For example, classification modeling can effectively classify power system anomalies, and regression methods can predict energy consumption with high accuracy.
By training machine learning models on labeled datasets, organizations improve prediction accuracy, ensuring reliability in energy management.
Unsupervised Learning for Anomaly Detection
Unsupervised learning methods are essential for algorithms that detect unexpected anomalies in energy usage patterns. Using clustering algorithms makes the opportunity to detect anomalies greater, and dimensionality reduction techniques make these greater.
These predictive models adapt rapidly to new operational environments. First, they can automatically flag anomalies without requiring prior human labeling of data, so they can respond more quickly when something goes wrong.
Reinforcement Learning for Grid Optimization
Reinforcement learning provides exciting new techniques to improve grid performance. Through the utilization of adaptive control strategies, power grids are able to react in real time to changing conditions.
Agent-based models allow us to simulate operations, test and implement feedback loops, providing for a dynamic and ongoing process to improve. Controlled training environments for AI agents help them learn to avoid dangerous or inefficient behavior, making them more efficient and reliable.
While machine learning applications hold great promise for tackling power system complexities, they bring new challenges.
AI in Solar Energy Systems
AI technologies go a long way in maximizing the efficiency of solar energy systems, giving meaningful returns to energy producers and consumers. With AI-powered real-time monitoring, solar panel performance can be optimized at any given moment so that they produce the maximum amount of energy possible.
For example, AI algorithms are being used to process weather forecasts to improve solar energy generation prediction, enabling improved energy dispatch. Implementing machine learning models that evaluate historical production data further refines these forecasts, leading to smarter decisions in energy management.
AI for Solar Power Forecasting
AI can be used to predict solar energy generation based on changing weather conditions through neural networks. Using or developing supervised machine learning models, these models look at historical performance, drastically improving forecasting accuracy.
Additionally, systems can dispatch energy in response to solar generation forecasts, allowing for a more flexible energy grid. Intuitive and informative interfaces help bring all stakeholders along by providing key forecasting information and helping create a transparent decision-making process.
Optimizing Solar Panel Placement with AI
AI is really good at figuring out the best places for solar panels, based on an analysis of geographic data and surrounding conditions. In addition, simulation models calculate the best installation scenarios, creating efficient layout options that maximize exposure and decrease unwanted shading.
Algorithms can quickly calculate possible shading, orientation, tilt, etc., to make sure that installations capture as much energy as possible. These decision-support tools help them plan community-scaled solar projects, setting up smoother, more effective projects.
AI-Driven Maintenance for Solar Farms
AI further accelerates and informs maintenance activities for solar farms, minimizing downtime and driving more efficient maintenance. For solar projects, predictive maintenance strategies use AI to continuously monitor panel health and detect potential issues before they become costly blackouts.
AI-powered management systems direct resources where they are needed most, making maintenance proactive and responsive.
AI for Smart Grid Management
AI enables improvements to smart grid technologies that help us manage our energy infrastructure more effectively and efficiently. Through AI solutions, we will be able to reduce redundancies, distribute energy more effectively, and develop more robust communication between grid elements.
For example, AI uses data from smart meters to help predict energy demand and distribution with greater accuracy.
Demand Response Optimization
AI plays a role even further downstream, helping to better understand consumer behavior to optimize demand response programs. With data from smart meters, machine learning models are able to effectively predict peak demand periods and manage energy accordingly.
Automated systems can use these forecasts to proactively adjust energy consumption, creating a more balanced load on the grid. AI-powered user engagement tools like Uplight’s Wave platform drive higher enrollment and activity levels in demand response programs. In turn, consumers save on their energy bills.
Smart Meter Data Analysis
When used alongside AI, analyzing data from millions of smart meters can provide these utilities with truly actionable insights. Algorithms are able to quickly detect anomalies in consumption patterns, alerting operators of potential problems before they materialize.
With visualization tools, this data can be presented clearly, quickly conveying the information to decision-makers. These predictive models can help forecast energy usage in the future, taking the guesswork out of long-term capital planning and resource allocation.
Enhancing Grid Security with AI
AI-powered cybersecurity solutions will be crucial in fighting to keep our power grid’s infrastructure safe. In the event of an attack, machine learning models automatically detect and respond to cyber threats in real time, safeguarding an organization’s operations.
Systems meant to monitor and analyze vehicle traffic data can better spot unusual or dangerous activity, and AI-enabled protocols help improve incident response and recovery efforts.
Challenges and Limitations
Integrating AI technologies, particularly generative AI, into power systems presents a host of potential challenges that must be addressed for effective implementation. Recognizing these challenges is critical for realizing the full promise of AI capabilities in the energy space.
Data Availability and Quality
It is important to have high-quality data in order to train effective AI models. Without quality, accurate and reliable data, AI applications will be working with a handicap to produce the best results possible. Utility-owned equipment can improve the quality of data by deploying smart sensors and Internet of Things (IoT) devices to allow for real-time monitoring.
Examples of these common challenges might be data silos and database inconsistencies. As such, collaboration between utilities and data providers is essential to streamline data quality and accessibility.
Computational Costs
Deploying AI technologies comes with big dollar signs attached. The cost of computational power can be significant, particularly for large-scale deployments. Yet, by far, the costs of AI solutions, like increased efficiency or predictive maintenance, are eclipsed by the benefits.
Unpacking economical solutions, such as utilizing cloud computing, can go a long way in reducing costs. Further identifying funding opportunities for early-stage AI-supported research and development can help accelerate AI adoption throughout the energy management ecosystem.
Security and Privacy Concerns
AI applications in power systems introduce new security vulnerabilities, such as the risk of malicious cyberattacks on critical infrastructure. Privacy implications flow from this massive data collection as well. To safeguard against misuse of data, regulation is necessary to create standards for AI-powered technologies.
By promoting transparency in AI algorithms, governments can build trust and confidence among their stakeholders that their data will be used and protected responsibly.
Regulatory Hurdles
Another critical barrier is regulatory challenges that can stifle AI innovation in power systems. Updated policies are necessary to accommodate evolving AI technologies. With intentional engagement, these challenges can be overcome through the thoughtful collaboration of regulators and the industry stakeholders they oversee.
Looking at real-world examples, or case studies, where regulatory frameworks have evolved to meet these challenges head-on can offer useful insights.
Future Trends in AI for Power
The adoption of artificial intelligence (AI) in power systems is transforming our approach to managing electricity. Emerging trends highlight powerful opportunities to improve security, enhance grid stabilization, and unlock higher performance in clean electricity generation.
Edge Computing in Power Systems
Edge computing, the practice of processing data as close to the source as possible, is transforming the way we handle information. It’s this machine-first approach that drives faster insights, increasing the speed of insight to action by minimizing decision-making latency.
With AI deployed at the edge, you can start translating that intelligence into real-time power management outcomes. For example, a major utility provider is able to track grid status and react to outages in real-time.
Creating standards that integrate edge computing and traditional power infrastructure paves the way for a smooth transition. As evidenced by successful case studies, like those found from the Department of Energy’s smart grid initiatives, edge computing is proving to increase operational efficiency and reliability.
AI-Based Cyber Security Solutions
AI technologies are central to strengthening cybersecurity in our power systems. With the help of machine learning models, organizations can better predict, detect, and respond to cyber threats.
Deploying AI-supported incident response systems enables faster recovery, reducing the impact of downtime during attacks to a minimum. Creating awareness programs underscores the importance of cybersecurity in AI applications, ensuring that all stakeholders understand the risks and mitigations involved.
Digital Twins for Power System Simulation
Digital twin technology creates dynamic, virtual replicas of power systems, enabling simulations and predictive analysis of their operation and performance. With the help of AI algorithms, these digital twins can exceed optimizations to operations and enable predictive analysis.
Scenario testing becomes exponentially more fruitful, enabling better planning, resource allocation and decision-making. Tools that allow stakeholders to engage with digital twins in an interactive way can be illuminating, yielding new insights to support more informed decision-making.
Transition to Decentralized Control
The transition to decentralized control in power management represents the next step in a rapidly evolving paradigm in management of energy systems. This change helps increase coordination between distributed energy resources such as rooftop solar and community wind.
In the process, it fosters a more resilient and efficient grid. Transformative AI solutions allow for much deeper coordination. This will give us the opportunity to adjust energy flows in real-time, balancing energy demand with new supply dynamics.
AI for Distributed Generation
AI technologies are key to ensuring the best possible integration of these distributed energy resources. Algorithms control energy flows between local generation sources and consumption points in real-time, dynamically optimizing and stabilizing the system.
This productive consumption of energy goes a long way toward eliminating energy waste. Creating back-end systems that improve their interaction with the grid makes things easier for everyone. Decision-support tools provide assistance for the planning and installation of distributed generation projects.
They help utilities manage their data and predict future energy needs, making the process more systematic and informed.
Microgrid Management with AI
AI extends beyond the grid, revolutionizing microgrid management to drive efficiency and guarantee reliability. Through real-time monitoring systems, we will have fast and precise control capabilities over our microgrids, with the ability to respond to changes in energy demand in seconds.
Predictive models offer increased reliability, predicting where changes might disrupt plans, enabling action before the moment something drastic needs to happen. Frameworks provide a foundation for smooth coordination of microgrids with the rest of the grid.
This allows for energy distribution to be both flexible and strong.
Compare AI with Traditional Approaches
AI-driven solutions in power systems provide unique benefits compared to traditional approaches. Traditional approaches fall short because they’re based on rigid rules and historical data, which constrain their ability to adjust to evolving conditions. Unlike AI, it cannot sift through millions of real-time data points to strategically shift operations quickly and seamlessly to achieve optimal performance.
This flexibility means AI can help to adjust for peaks and drops in energy usage, creating a more resilient power grid.
Cost Analysis
A robust cost-benefit analysis indicates that upfront AI investments are considerable. The ROI is huge—not just financially, but for research as a whole. Utilities that implement AI to proactively manage energy with the technology can see fantastic cost savings.
Thanks to greater efficiency, they usually experience savings of at least 30%. Long-term financial benefits come from lower operational costs through better predictive maintenance leading to less downtime and longer life of equipment.
In modeling future cost savings, we demonstrated that as AI technologies develop, the financial benefits will keep growing.
Efficiency Gains
With the right support and leadership, AI integration has already produced impressive efficiency gains. For instance, AI algorithms minimizing wasted energy by optimizing grid operations can save 15 percent of energy consumed. Such improvements are the result of more effective demand forecasting and resource allocation.
Metrics such as reduced energy losses and improved load balancing quantify these benefits, highlighting AI’s role in sustainable energy management.
Risk Mitigation Strategies
AI contributes to power system stability with proactive risk management. By predicting equipment failures before they happen, AI prevents unplanned outages. Having AI-driven analytics to constantly examine the health of a system means creating an overall more reliable system.
By utilizing frameworks that integrate AI into an overall risk management strategy, agencies can prevent these problems before they occur, protecting our vital infrastructure.
Case Studies of AI Implementation
AI is transforming our electric power systems to make them more efficient and reliable. By examining case studies of generative AI support systems with proven success, we can learn from the thought processes that have brought about meaningful change.
Successful AI Projects
One example worth examining further is the use of AI in Pacific Gas and Electric’s grid management efforts. They implemented machine learning to anticipate outages, allowing them to cut downtime by 50%. Tools such as neural networks and predictive analytics were crucial to this shift.
It was a challenge, for sure, with integrating data across multiple source systems, but that was all worked through collaboratively with our tech partners. The benefits were clear: improved customer satisfaction and reduced operational costs.
A third case is the company-wide adoption of AI and machine learning by Siemens in smart grid technology. They started by looking at real-time analysis data and determining where energy could best be distributed.
The methodologies used even incorporated machine learning algorithms to predict energy usage in the most precise way. By tackling the problems that came with legacy systems, Siemens improved their grid’s ability to adapt and withstand disruption.
The result was a more reliable energy supply at lower cost and with less emissions.
Lessons Learned
All of these projects are excellent examples of what can be accomplished when stakeholders come together. By involving all stakeholders—from utilities to technology companies—up front, AI will be better positioned for successful deployment.
Best practices from early implementations suggest beginning with pilot programs that test the value of AI applications before deploying at full scale. Perhaps the most common pitfall is not realizing the importance of data quality and preparation.
Moving forward, their future projects can focus on increasing transparency regarding how AI makes decisions to create trust with users.
Conclusion
AI is one piece of the puzzle in the evolving power systems landscape. It increases efficiency, improves grid management and the integration of renewable energy resources. With the help of machine learning, we’re able to better predict demand, optimize resources, and streamline operations. The move away from centralized to decentralized control really is breaking new ground that makes smarter grids and more efficient energy distribution possible. Real-world use cases illustrate how companies are winning with AI, boosting productivity and reducing expenses.
It’s clear that as we march ahead, embracing AI’s development and implementation will be essential. These challenges won’t slow down the momentum, but will continue to spur creativity and innovation. Continue to keep an eye on these trends and think about how AI can revolutionize your approach to energy management. Don’t wait—begin your journey today and discover how AI can transform your power systems.
Frequently Asked Questions
What is the role of AI in power systems?
AI has the potential to enhance operational efficiency and reliability in electric power systems, leveraging big data analytics to forecast equipment failures and improve the efficiency of energy delivery in the future power grid.
How does machine learning apply to power systems?
Machine learning algorithms, particularly generative AI systems, have the ability to recognize patterns in data, allowing for more effective forecasting and maintenance strategies to be implemented in electric power systems, minimizing downtime and operating expenses.
Can AI improve solar energy systems?
AI increases efficiency in the power sector by optimizing energy production and consumption within solar systems. Utilizing generative AI, it predicts weather patterns and optimizes settings, ensuring systems run at peak output for improved overall efficiency.
What is smart grid management with AI?
AI’s role in smart grid management, particularly through generative AI systems, allows for real-time data analysis and enables a more responsive distribution of electricity. Not only does it improve grid reliability, but it also facilitates the seamless integration of renewable energy sources.
What are the challenges of implementing AI in power systems?
These challenges, including data privacy concerns and high implementation costs, along with a shortage of skilled personnel, must be addressed to ensure the successful integration of AI systems into the power sector.
What are future trends in AI for power systems?
Looking ahead, advances in automation, predictive analytics, and generative AI support systems, alongside the convergence with renewable energy generation, will be key trends to watch in the future power grid.
How does AI compare to traditional approaches in power systems?
AI, particularly through generative AI support systems, is proving superior to traditional approaches by offering data-driven insights, faster decision-making, and enhanced predictive maintenance, ultimately creating improved productivity and delivering significant cost benefits.
What's Your Reaction?
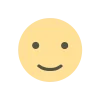
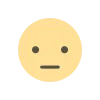
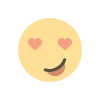
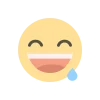
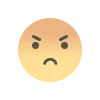
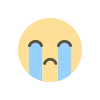
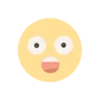