The Application of Mathematics in AI Technologies
Explore the unique application of mathematics in artificial intelligence with our comprehensive PPT, featuring insights beyond standard presentations.
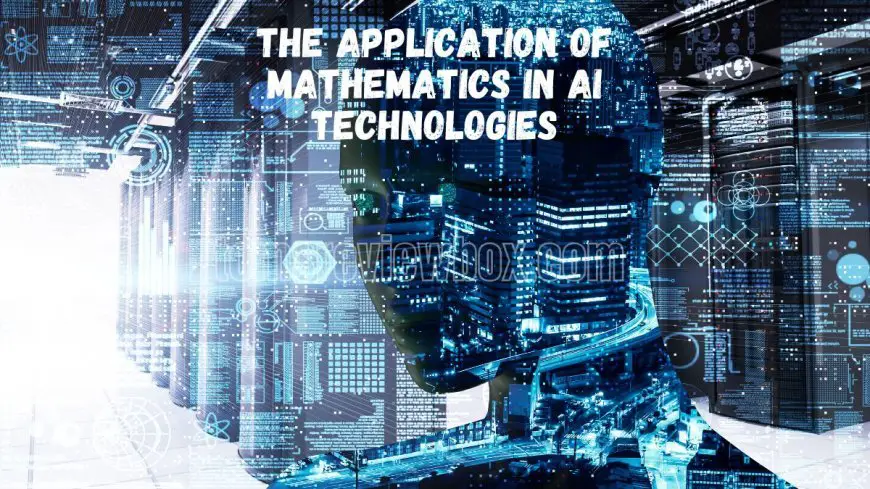
The rigorous application of mathematics to AI has proven essential to developing robust, intelligent systems. Math underpins the algorithms that allow machines to learn from data and make intelligent decisions.
Statistics help to analyze trends, establishing an indispensable foundation for artificial intelligence. At the same time, linear algebra undergirds neural networks, rooting their operating principles in deep mathematical foundations.
Calculus, for example, is needed to optimize algorithms and probability theory to make predictions. An understanding of these principles will improve your understanding of AI and lead you to possibilities for new, creative solutions.
In this post, we will explore various mathematical applications in AI, illustrating how they shape the technology we interact with daily.
Key Takeaways
- This is why it’s so important to understand the mathematical foundations of AI. Key areas like linear algebra, probability, and calculus are essential for grasping how AI algorithms work and making informed decisions.
- Linear algebra is arguably the most important branch of mathematics in applications to neural networks, providing the foundation for data representation and optimization. Becoming familiar with these vectors and matrices will help you better understand what is happening behind the scenes in AI computations.
- Probability and statistics are key to understanding how to make predictions in AI. Focusing on these ideas will make you a better modeler and help you with model validation.
- Calculus helps AI professionals optimize algorithms. Just like with backpropagation, knowing how math applies in practice, such as how you can use gradient descent to train effective models, is invaluable.
- Differential equations provide powerful, interpretable models of dynamic systems in AI, enabling the prediction of long-term behavior. This type of mathematical understanding is necessary for applications such as reinforcement learning to be used effectively.
- Discrete mathematics plays an important role in this field. Having a solid understanding of combinatorial structures and graph theory will greatly improve your problem-solving capabilities with AI.
What is AI's Mathematical Foundation?
Indeed, mathematics gives us the very foundation for the limitless possibilities of artificial intelligence (AI). These foundational concepts such as linear algebra, calculus, and probability will be the backbone for many AI methodologies and algorithms.
These areas of mathematics do more than just enable analysis of data — they improve model accuracy and increase the integrity of AI-driven decision making.
1. Linear Algebra's Role in Neural Networks
Linear algebra is especially important in AI today, particularly in neural networks, designed to replicate the structure of the human brain. With the use of vectors and matrices, data is represented and manipulated in a way that simplifies computations and allows AI practitioners to efficiently model complex environments.
From a practical standpoint, for instance, 80% of the machine learning algorithms used today derive from linear algebra. With linear transformations, data can be represented efficiently, instead of vertically with high dimensions, making the network deliver its best performance through mathematical operations on these structures.
2. Probability for AI Predictions
Probability theory is a fundamental part of AI, as it’s the basis for making predictions and analyzing uncertainty. It enhances decision-making across all disciplines.
This is true for NLP or speech recognition for example, areas where probabilistic models such as Hidden Markov Models excel. Such models have been useful not only in tasks such as part-of-speech tagging, but in generating coherent and salient language.
3. Calculus and Optimization in Training
Calculus, specifically differential calculus, has fundamental importance in AI by helping optimize algorithms through training. Specifically, it aids in optimizing weights and biases in neural networks, ensuring efficient convergence and processing.
With an understanding of derivatives comes an understanding of model behavior and better, more robust solutions can be found.
4. Differential Equations in Dynamic Systems
In AI, differential equations are used to model complex, dynamic systems, allowing us to simulate and understand real-world phenomena. They are essential to understanding progress over time and enabling breakthrough impacts to come from AI solutions.
5. Statistical Learning and Generalization
Statistical learning underlies continual improvements in the actual performance of AI models. Methods that guarantee generalization minimize bias, maximizing the bias/variance tradeoff, which makes the model more trustworthy.
Linear Algebra in AI Explained
From Google PageRank to ChatGPT, linear algebra is the hidden structure behind artificial intelligence today. At its core, linear algebra is about manipulating vectors and matrices to effectively analyze and represent data. If you’re looking to understand these concepts, it is a must.
Here are a few fundamental elements, and how they’re used to create AI applications.
Vectors and Matrices in AI
Component |
Role in AI Applications |
---|---|
Vectors |
Represent data points and features |
Matrices |
Manage multiple data points and relationships |
Vectors play a crucial role in AI computations, allowing us to represent data in a way that machines can process. To take an example from natural language processing, words are commonly encoded as vectors—arrays of numbers that summarize the meaning and relationships between the words.
That’s because matrix multiplication is at the heart of all neural networks. It’s a beautiful linear algebra contraction that takes inputs and weights and produces outputs.
Linear Transformations in Machine Learning
Linear transformations change the dimensions of the data, often improving the performance of the model. By lowering the dimensionality of a dataset using transformation, we simplify the model.
This methodology has helped us to find the balance of keeping critical details all while streamlining the process. Such simplifications improved model interpretability, helping to inform the best possible decisions.
Eigenvalues and Eigenvectors Significance
Eigenvalues and eigenvectors hover all around the data analytic sphere. In PCA (and other similar techniques), they capably serve to reduce dimensions while retaining maximum variance.
This is key not only for enhancing computational efficiency, but for keeping AI systems reliable and grounded.
Probability and Statistics in AI
AI is grounded in probability and statistics, equipping us with the tools to understand uncertainty and power data-driven decisions. These theoretical ideas are super important when testing AI models, making sure they’re operating at their best when applied to the real-world scenario.
By embedding statistical approaches to AI algorithm development, practitioners can improve the robustness and reliability of the model. As an example, machine learning algorithms use statistical techniques to learn from data and make predictions that accurately represent real-world situations.
Bayesian Networks Explained
Bayesian networks are powerful structures in AI that represent variables and their conditional dependencies through directed acyclic graphs. They use Bayesian inference to reason under uncertainty, allowing AI systems to update beliefs based on new evidence.
Applications of Bayesian networks span various domains, including medical diagnosis and risk assessment, demonstrating their versatility in handling complex data.
Hypothesis Testing in AI
- Formulate a hypothesis.
- Collect data.
- Analyze data using statistical tests.
- Draw conclusions.
- Validate findings.
Hypothesis testing is critical to validating the models that will be developed for AI. It provides the basis for deciding whether observed effects exceed the noise of random sampling variability, powerfully informing choice, risk management and regulatory decisions.
Statistical significance helps us understand when AI outcomes can be deemed reliable and therefore actionable.
Regression Analysis in AI
Regression analysis is one of the most basic and important techniques of predictive modeling, used to understand the relationship between variables.
Regression, including linear and logistic regression, is used for predicting future trends or behaviors, classification, and more in AI. This approach explains how various factors impact outcomes, yielding knowledge critically important in improving AI models.
Calculus and Optimization Techniques
Calculus is one of the most important tools in AI, in particular when it comes to optimizing the models used. Key concepts like derivatives and integrals play a crucial role in helping us understand how changes in input affect outputs. The power of calculus to optimization is significant.
When we combine calculus with optimization techniques, we can further refine AI models, helping them make more precise predictions. Optimization techniques help to improve model accuracy by finding the optimal parameters. This has a direct effect on performance.
Gradient Descent Methods
The gradient descent algorithm is foundational to the training of many types of AI models. It uses a feedback loop that iteratively adjusts parameters to minimize errors, steering the model intelligently toward peak performance.
Optimizations such as stochastic gradient descent and its cousin mini-batch gradient descent help to make things more efficient by working with smaller batches of data. This technique alleviates computational burden and accelerates convergence, proving invaluable under big data.
Through minimization of these loss functions, gradient descent enables model predictions to reflect real-world outcomes, increasing model reliability.
Convex Optimization in AI
Convex optimization addresses a special class of problems in which all local minima are global minima. This property is key in AI, because it makes the search for optimal solutions straightforward.
Convex functions’ well-behaved nature means algorithms will always and easily converge to optimal solutions, making the algorithms converge reliably. Real-world applications to machine learning, such as support vector machines and logistic regression, showcase the brilliance of convex optimization.
This is a powerful approach to massively improve model performance.
Lagrange Multipliers Application
Lagrange multipliers are the magician’s wand in constrained optimization, giving us the ability to find maxima and minima subject to constraints. In practice, this plays an important role in model training, where it forces balance between performance objectives and resource constraints.
Applications to real-world resource allocation problems in neural networks show their practical importance.
How Differential Equations Impact AI
As we’ve seen through several examples, differential equations are fundamental to the creation, training, and operation of AI applications. As a result, they are powerful tools to model behaviors and predict outcomes, providing the window necessary to understand the complex dynamic behind disaster relief. By integrating these equations, AI can more effectively respond to shifts and refine its decision-making mechanisms.
Modeling Dynamic Systems
Dynamic systems are fundamental to AI modeling because they capture processes that change over time. These systems are typically modeled with differential equations, which effectively highlight the interconnectedness and dynamic behavior of variables.
Approaches such as state-space representation and feedback loops offer a robust structure for these models. For example, when an AI system identifies a new trend emerging in the data, it can immediately update its forecasts accordingly.
By allowing for this dynamic model, it significantly enhances its ability to adjust to new environments.
Applications in Reinforcement Learning
In reinforcement learning, differential equations play a crucial role in defining the behavior of agents. They play an important role in determining how agents learn and adapt via interaction with their environment.
Algorithms such as Q-learning can use differential equations to include continuous state spaces. This thoughtful and creative approach makes for remarkably richer learning experiences.
Through this dynamic modeling, agents can better plan their maneuvers through complicated situations and execute their actions in a more time-effective manner.
Stability Analysis with Differential Equations
Stability analysis is essential for guaranteeing that an AI system will operate in a predictable manner as time progresses. Differential equations allow us to evaluate the stability of these systems.
This allows us to evaluate whether their behaviors are likely to converge to a state we want or diverge. Techniques like Lyapunov stability offer a deeper understanding of system dynamics, enabling robust performance, which is crucial when deployed in real life.
Discrete Mathematics' Role in AI
Discrete math is the bedrock of artificial intelligence, equipping curious minds with profound tools and frameworks to tackle complex problems. Its central fields — logic, combinatorics, and set theory — each provide distinct, vital contributions to AI’s power and potential.
Logic and Proofs in AI
Logic is fundamental for creating algorithms that mimic human reasoning. Predicate logic helps in understanding relationships and properties of data. Induction and contradiction are essential techniques for proving algorithms correct.
Formal discrete structures such as logic are essential to the AI reasoning pipelines. It allows complex systems to derive logical decisions stemming from fundamental principles. Proofs help to ensure the proven correctness of algorithms, assuring confidence in their reliability and performance.
Combinatorics in AI Algorithms
Combinatorics, the mathematical study of arrangements and combinations, is a cornerstone of AI algorithm design. It improves the bottom line of algorithms through more optimized solutions.
As an example, combinatorial optimization techniques are often employed in resource scheduling problems, where calculating an optimal arrangement can drastically increase efficiency. Beyond understanding AI’s effects, combinatorial structures, such as graphs, underpin many complex AI challenges, including network connectivity.
Set Theory in AI
Set theory is essential for efficaciously structuring data used in AI. It offers a powerful mathematical framework to precisely define arbitrary collections of objects, an extremely important workhorse in data management.
Set operations, like unions and intersections, are widely used in AI algorithms to efficiently manipulate and analyze data. Concepts in set theory provide better comprehension of relationships between data points, which leads to decreased bias in AI models.
Emerging Mathematical Trends in AI
Mathematics is indeed a central theme to the entire discipline of artificial intelligence, and has driven much of its development and success. Whether you are conducting research, building AI applications, or creating policy, an understanding of these emerging trends is essential. Emerging mathematical concepts have the potential to build AI methodologies that go beyond doing more with less.
Topological Data Analysis
Topological data analysis (TDA) is a new approach to data that is concerned with its shape. This is important because it allows you to find underlying trends in complicated, multi-dimensional table-like data. For example, using TDA, businesses can uncover clusters in their consumer behavior data, enabling them to customize their marketing tactics to target specific customer segments.
AI more generally employs topological strategies to improve understandings of data sets across sectors such as public health and medicine. This method can reveal interactions within the patient data that more traditional methods may miss.
Category Theory Applications
Category theory provides a language to unify disparate mathematical concepts. In this way, it provides an organizing principle for relationships in AI, from the engineering perspective to the societal implications. Category theory offers a powerful framework to simplify and standardize the connection between many different AI models.
This trend complicates efforts to build safe, reliable systems. This systematic way of thinking is especially valuable in machine learning, where identifying important dependencies among variables is essential for maximizing predictive performance of a model.
Information Geometry
Information geometry examines the differential geometric structure of statistical models. This fragile space is particularly important and fragile for AI. It hones algorithms to a fine point by providing a deeper understanding of how the data informs the probability distributions.
For example, it can exploit neural networks by accelerating their convergence rates. AI applications in natural language processing using information geometry further improve models that decode intricate language details.
Conclusion
Mathematics continues to power artificial intelligence, powering its rapid growth and drive for innovation. If they don’t understand their work would be seen at each mathematical concept. Linear algebra underpins the way we handle data, and probability underpins how we make decisions in uncertainty. That’s where the beauty lies—in the fusion of these technical elements to create powerful tools that address the most pressing real-world problems. As AI continues to grow and develop, staying informed on emerging trends in mathematics will help you unlock new math learning potential!
Get ready to evolve your understanding of math as it applies to AI. Learn more about how math fuels AI, and how you can get in on the action. The further you go, the better equipped you’ll be to harness these tools to break new ground. So, join us as we continue this important discussion of how math and AI can ignite new possibilities.
Frequently Asked Questions
What is the role of mathematics in artificial intelligence?
Mathematics offers the critical underpinnings for AI algorithms, from statistics and optimization to linear algebra and calculus. It aids in model formulation, data analysis, and optimization, empowering machines to learn and make optimal decisions effortlessly.
How does linear algebra apply to AI?
Linear algebra is very important in AI. In AI, you are often dealing with data structures, especially matrices and vectors. It enables fast operations over neural networks, image processing, dimensionality reduction, etc.
Why are probability and statistics important in AI?
Applications AI systems, powered by probability and statistics, are used in diverse fields to make predictions and decisions in uncertain environments. They are important for understanding data distributions and training models properly.
What is the significance of calculus in AI?
Calculus is at the very foundation of AI, specifically for optimizing AI algorithms. It gives possible ability of gradients calculation, gradients are used in training models to minimize error and maximize performance.
How do differential equations influence AI?
Differential equations are used extensively in modeling dynamic systems and processes. In AI, they’re applied mostly to predict things that will happen over time, being utilized primarily in fields such as robotics and control systems.
What is the role of discrete mathematics in AI?
Algorithms and data structures in computer science are based in discrete mathematics. It is key in terms of understanding graph theory, combinatorics, logic, which are all fundamental in AI development.
What emerging mathematical trends are shaping AI?
Among these trends are exciting new developments in topological data analysis and new machine learning optimization methods. Taken together, these innovations significantly expand AI’s capacity to analyze complex data and increase the accuracy of predictions.
What's Your Reaction?
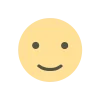
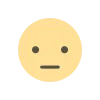
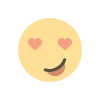
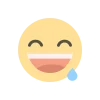
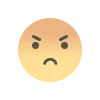
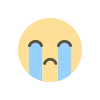
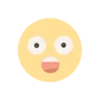