10 Key Applications of Machine Learning in E-commerce
Discover 10 key applications of machine learning in e-commerce that enhance customer experience and boost sales effectively.
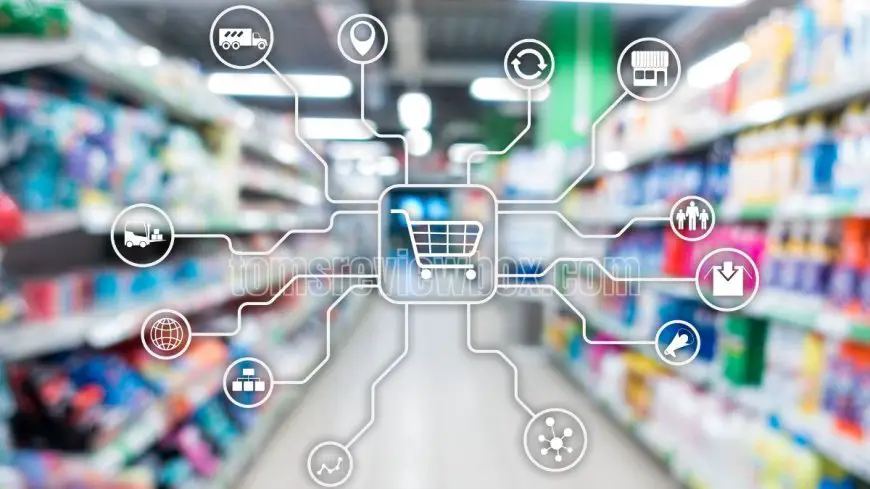
It’s critical value of machine learning that ecommerce companies tap to create better experiences for customers while making their businesses more productive. By automatically analyzing massive amounts of data, machine learning algorithms are able to more accurately predict consumer behavior, as well as personalize recommendations to consumers and optimize inventory management.
Take a look at how well this works every time you visit a site that recommends products based on your past purchases or browsing behavior. These systems make the shopping experience faster and more efficient, while simultaneously maximizing revenue opportunities with precision marketing tactics.
By understanding how these technologies actually work, you can learn how they’ll most transform your business. I’ve written this post to explore different machine learning techniques that are being applied in ecommerce right now. Not to mention that you’ll walk away with practical insights that will help you harness this technology in your own work.
Key Takeaways
- Machine learning, artificial intelligence’s advanced counterpart, is a crucial component of e-commerce. It strengthens business intelligence by quickly analyzing massive datasets, resulting in more personalized customer interactions and increased overall efficiency. While machine learning takes some time to implement and learn, it can greatly accelerate your business ecommerce success.
- Personalization has become a requisite in today’s hyper competitive landscape. Use ML to create intelligent product recommendations and refine social media or search marketing campaigns. These new capabilities will increase customer interactivity and engagement, leading to increased sales conversion rates.
- Automating other e-commerce processes, like running targeted marketing campaigns or identifying fraud, creates a more streamlined workflow. This saves time and minimizes errors, resulting in increased customer trust and satisfaction.
- Constant monitoring and refinement of machine learning models are essential to keep pace with shifting consumer behaviors and market conditions. With continual updates, you’ll make sure your strategies stay on the cutting edge and ahead of the competition.
- Address these issues before they become obstacles by ensuring a cohesive interaction of machine learning tools and current processes. Consider data privacy an essential quality and architect for long-term scalability from day one.
- Don’t get left behind by new technology! Look into new developments in machine learning, such as reinforcement learning and deep learning, to improve customer engagement and support better business operations.
What is Machine Learning in E-commerce?
Machine learning (ML) has quickly established itself as a vital component of artificial intelligence (AI) with distinct applications that enhance decision-making in the ecommerce industry. By analyzing large datasets, ML significantly contributes to creating better customer experiences through personalized interactions and operational efficiencies.
Alongside targeted email marketing campaigns, ML-based recommendation systems thrive on major ecommerce platforms. These systems boost upselling by suggesting specific products customers might prefer, ultimately increasing your average order value.
More importantly, AI and ML excel at predicting future trends and consumer behavior. Retailers can effectively forecast demand and identify emerging sales patterns, allowing them to align supply with customer needs while minimizing overstock.
ML could look at historical order information to improve delivery modes. Plus, it suggests the most cost-effective shipping methods, helping you distribute products on time and at low cost.
The ability to automate ecommerce operations is another crucial aspect of ML. GenAI-powered chatbots can cut customer service costs by up to 30% while simultaneously boosting revenue through tailored shopping experiences.
Moreover, machine learning solutions can identify outlier patterns, alerting businesses to potential fraud or anomalies in checkout workflows, which is essential for maintaining a secure online shopping environment.
How Machine Learning Transforms E-commerce
Machine learning technology is redefining the e-commerce landscape, powering tangible growth and efficiency. By analyzing user behavior and preferences, many ecommerce businesses can create personalized shopping experiences that resonate with customers.
1. Personalize Product Recommendations
One of the most significant ways is through product recommendations. To give an example, Amazon uses collaborative filtering and content-based filtering to recommend products to users based on their previous purchases. This approach improves the customer experience, and more than 80% of retail shoppers like AI-driven solutions that help them understand their product options.
By constantly updating user behavior data, businesses can make these recommendations even more precise over time.
2. Enhance E-commerce Site Search
Enhanced search performance enables more relevant and precise product listings. Intelligent algorithms and natural language processing make it easier to interpret customer queries.
By adopting visual search features, you give users the ability to search using images, transforming the search process into an intuitive and efficient experience.
3. Automate Marketing Campaigns
Machine learning makes marketing more efficient by allowing businesses to segment their audiences and focus on the most promising leads. Automating email campaigns triggered by recipient interactions guarantees that the messaging is more personalized and relevant to recipients.
Predictive analytics can help predict the performance of specific campaigns before launching them, allowing businesses to pivot strategies before they go live.
4. Improve Fraud Detection Systems
On the security side, machine learning technology is significantly improving fraud detection ability in the ecommerce environment. By using machine learning to visualize customer transaction patterns, ecommerce platforms like Stripe can quickly detect anomalies and safely block fraudulent transactions.
Real-World Machine Learning E-commerce Examples
Now, machine learning technology has emerged as a central pillar of ecommerce, allowing many ecommerce businesses to personalize customer journeys, streamline operations, and make informed decisions. Top ecommerce platforms utilize the latest machine learning solutions to inspire purchase decisions and connect users to brands in impactful ways.
Personalized Recommendations in Action
E-commerce leaders like Amazon and eBay have long used these personalized recommendation systems to great success. Take, for example, Amazon’s recommendation system — powered by artificial intelligence — that drives 35% of the company’s sales.
This is important because a site that provides personalized product recommendations can improve its customer repeat rate by 56%. These recommendation engines leverage data, studying user behavior to understand patterns and preferences, powering personalized recommendations that connect with the unique tastes of each shopper.
Behind the scenes, machine learning allows these companies to constantly improve the accuracy of these recommendations, ultimately increasing user satisfaction and engagement.
Localized Shopping Experiences Explained
Machine learning doesn’t just help retailers customize shopping experiences. Companies can leverage geographic data to take personalization a step further.
By integrating cultural relevance into their product recommendations, companies increase the likelihood of satisfying customers. Local preferences can help plan inventory, making sure that products best suited to regional tastes are most available.
Dynamic Pricing Implementation
Further showing the power of machine learning, real-time price adjustments are perhaps its most impressive benefit. Retail companies including Walmart use dynamic pricing to capture the highest possible revenue.
Customer reactions are unpredictable—being open about what’s going on with pricing is key to keeping consumers’ trust.
Inventory Optimization Strategies
Predicting stock levels with machine learning provides more accurate inventory forecasts. There are always outliers.
Enhanced by machine learning, techniques for demand forecasting allow businesses to make data-driven decisions while maximizing efficiency.
Violation Detection Systems Showcased
Machine learning is the backbone of powerful policy violation detection, ensuring continued compliance and preserving public trust.
By requiring companies using these systems to proactively address problems, FHWA ensures the integrity of our operations.
Best Practices: Implement Machine Learning
Implementing machine learning technology in e-commerce isn’t a simple task. These four points underscore important steps toward meaningful implementation, emphasizing the need for ongoing iteration and improvement in the context of ecommerce strategies that align with the organization’s business objectives.
Define Clear Objectives First
Setting concrete, measurable objectives is the most important first step for any machine learning project. You’ll want to tie these objectives to wider business strategies, making sure that everyone on the team knows the why behind every project.
Second, to improve customer retention develop specific metrics. Set a realistic, measurable goal as in a specific percentage of increase in repeat purchases and work toward that. Constantly revisit and revise these goals in light of actual performance results, enabling a nimble response to shifts in the market.
Prioritize High-Quality Data Collection
Accurate and relevant data is the bedrock of successful machine learning models. By implementing rigorous data cleaning procedures, the quality of our data can improve immensely.
It’s equally important to think about keeping within data privacy regulations during the data collection itself. Utilizing varied data sources such as customer feedback and purchase history can go a long way to deeply inform your models. This method results in better predictive accuracy.
Select Suitable ML Models
Selecting the appropriate machine learning model is critical. Test out various models to identify or tailor the most effective one for your individual use cases, keeping in mind aspects such as data type and quantity.
By testing a variety of models, you can find the most effective solution that meets your business needs.
Customize User Experiences
Personalization is a great way to boost customer loyalty. Personalize interfaces and experiences.
Learn what works best for each user and automatically modify the experience with adaptive algorithms that take cues from user interactions. This could involve targeted marketing messages based on browsing history, improving overall customer satisfaction.
Continuously Improve Models
Set expectations for how and when you will evaluate and update your machine learning models. Introduce new data to make them dynamic and evergreen, track performance metrics to identify needs for improvement.
Shifting the locus of innovation to your team promotes a culture of innovation that embraces experimentation, which is important for future success.
Machine Learning's Impact on E-commerce Trends
Beyond automating internal processes, machine learning technology is transforming the entire e-commerce industry, creating new opportunities and expanding customer bases. This technology enhances personalized discount offers and contextual shopping features, maximizing marketing strategy effectiveness and boosting operational efficiencies.
Forecasting Sales Accurately
Thanks to machine learning, businesses can now predict future sales trends with incredible accuracy. By leveraging historical data, seasonal trends, and an understanding of market conditions, companies can gain insight that helps drive successful inventory purchases and marketing strategies.
For example, a retailer might predict spikes in demand during the holiday season, allowing them to stock up but avoid overstocking. Regularly checking how forecasts are performing allows enterprises to correct course, making them more nimble.
Optimizing Omnichannel Marketing
This is particularly important in e-commerce, where seamless, unified marketing across all channels is vital. Machine learning allows brands to understand a customer’s previous interactions with the brand, enabling them to tailor messages to suit each platform appropriately.
Machine learning can help a brand track a customer’s path from social media to its website. It can then adjust its marketing strategy in response to the customer’s actions. By leveraging these strategies, brands can improve the experience to engage consumers, creating more relevant, personalized experiences at every touchpoint.
Estimating Customer Lifetime Value
Knowing your customer lifetime value (CLV) is key for profitable, long-term growth. Machine learning offers solutions to calculate and predict CLV by examining buying patterns and advanced engagement data.
This information has allowed companies to take action through effective retention strategies. Take, for instance, a subscription service that wants to create more personalized offers using first-party customer data to increase customer value over time.
Improving Delivery Route Efficiency
After all, improving efficiency in logistics translates to customer satisfaction with faster and more reliable deliveries. Machine learning algorithms optimize delivery routes, taking into account current traffic patterns and historical delivery times.
IoT-enabled real-time tracking systems monitor shipping performance and allow companies to lower their shipping costs by improving delivery speed.
Overcome Machine Learning Implementation Challenges
Although the benefits of implementing machine learning technology in the ecommerce industry are plentiful, so too are the challenges in integrating machine learning solutions. Recognizing these hurdles is key in optimizing ecommerce strategies to realize full benefits.
Integrate with Existing E-commerce Systems
One of the biggest hurdles is making sure it integrates seamlessly with other platforms you already have in place. You need to take stock of compatibility challenges upfront, laying out potential hurdles that could prevent fluid transitions.
Training staff on how new changes will impact their workflow is key to implementing these changes. Continuous monitoring of integration performance allows for timely adjustments, ensuring that the machine learning solution enhances operational efficiency without disruptions.
Address Scalability Concerns Effectively
Planning to scale up should start with your first practical implementation. Just as your e-commerce enterprise scales, so will the pressure on your machine learning implementations.
This allows you to do what-if growth scenario analyses to inform your infrastructure build-out to match future needs. Implementing cloud-based solutions provides the flexibility needed to scale efficiently, allowing you to adapt to increased traffic and data volume without compromising performance.
Ensure Privacy and Compliance
Data protection regulations are a hard reality that e-commerce companies must consider today. Following these rules when rolling out machine learning is essential.
Implement protocols for managing sensitive customer data and train staff on compliance guidelines. By continually reviewing and updating your policies, you can make sure you’re staying compliant with ever-changing regulations, protecting your business and your customers.
Improve Model Accuracy and Reduce Bias
You can’t implement machine learning if model accuracy is not high enough. Adopt strategies to check datasets for problematic bias and correct them before deployment to achieve equitable results.
By using varied datasets in the training process, you build models that provide more accurate results for different demographics. By continuously evaluating and understanding what areas of model performance need to be improved, smarter decisions can be made.
Future of Machine Learning in E-commerce
Artificial intelligence and machine learning have completely changed the game in e-commerce. It has the power to change the way companies interact with their customers and improve overall business effectiveness. Emerging trends showcase the potential of machine learning to enhance personalization and automation, allowing e-commerce platforms to deliver tailored experiences that resonate with individual preferences.
As you dive into these big changes, think about how they might change the way consumers experience changing the game.
Reinforcement Learning for Real-Time Decisions
Reinforcement learning is an important touchstone, as it is central to optimizing decision making and actions. Through predictive analysis of customer interactions, machine learning systems can continuously learn and adapt, making them more responsive to changing consumer behaviors.
For example, if a user consistently views certain categories of items, the algorithm can begin to modify recommendations to match. To the consumer, implementing such adaptive learning strategies seems like a guessing game, where what you’ve learned from previous decisions helps to shape what’s coming next.
Keeping a pulse on these applications helps us understand how to most effectively use them to ensure they’re helping increase customer satisfaction.
Deep Learning for Image Recognition
With the rapid development of artificial intelligence technology, deep learning algorithms are revolutionizing image recognition tasks within an e-commerce domain. These algorithms play a huge role in the product discovery process, making it easier for consumers to discover products using visual search tools.
Picture this — you take a photo of a dress and immediately get recommendations for similar dresses ready to buy. While you track the exciting things happening with deep learning, don’t forget to think about how this technology can help improve user experience and increase sales.
Machine Learning Market Research Integration
Now more than ever, it’s important to integrate machine learning with comprehensive market research to remain competitive. By using predictive analytics to decide what products to develop and market, retailers can ensure that they are producing products that consumers are looking for.
Processing insights obtained through machine learning improves the decision-making processes, helping businesses become more responsive to shifts in the market. Staying on top of these changes allows you to stay ahead of the curve.
E-commerce is consistently full of surprises.
Ethical Considerations for Machine Learning
In today’s dynamic, fast-paced environment of e-commerce, ethical use of machine learning applications is more important than ever. Enterprises are increasingly using AI to understand their customers, improve employee productivity, and predict outcomes. They need to place the responsible use of this technology front and center.
Ensuring machine learning ethical considerations keeps machine learning on the right path with company goals and objectives. At the same time, it honors the rights and dignity of constituents.
Data privacy remains a major issue. Customers have a right to know that their sensitive personal information is protected, and consent should be given freely, clearly and in a transparent manner. For instance, when an online retailer collects data to tailor recommendations, it is essential to inform customers about how their data will be used.
This cultivates a connection founded on trust, increasing customer loyalty in the long run. Having guidelines to ensure responsible, ethical, and inclusive AI use should be paramount in e-commerce. Businesses need to develop transparent, consistent training and invocation protocols for each algorithm.
This is to ensure that they don’t perpetuate bias or discrimination. For instance, if a machine learning model prioritizes certain demographics in product advertisements, it risks excluding others. Incorporating ethical guidelines will help to avoid such missteps.
Further, encouraging openness about how machine learning products work promotes customer trust and accountability. Providing transparency on what goes into the algorithm and how the technology operates can help educate consumers and remove the mystique that surrounds this technology.
For example, a company might explain that their recommendation system learns from past purchases to suggest relevant products, encouraging users to engage with the platform more confidently.
Conclusion
When you see how it can personalize customer experiences, increase operational efficiency, and drive greater sales, you start to understand the hype. Practical applications are proving its power—from bespoke shopping experiences to smarter stock control. In return, you receive invaluable insights from the best practices shared, helping you figure out how to rollout these new technologies seamlessly. By incorporating machine learning, you’ll be ahead of the curve and better prepared to face the unknown. As you plan for the future, consider the ethical implications of data usage. Work to ensure transparency and fairness. Once you have a firm grip on these areas, you’ll be empowered to decide strategically. Immerse yourself in the wonders of machine learning and unlock the secrets to e-commerce prosperity. Launch your machine learning strategy today and see your business truly take off.
Frequently Asked Questions
What is machine learning in e-commerce?
AI and machine learning technologies will similarly transform e-commerce by leveraging the potential of sophisticated algorithms and data. This machine learning technology enables businesses to understand and predict customer behavior, optimize their pricing strategies, and create tailored shopping experiences that enhance the overall shopping experience.
How does machine learning improve customer experience?
Machine learning technology plays a powerful role in the ecommerce industry by enabling personalized product recommendations, better search experiences, and more accurate predictions of customer preferences and intent. This results in a more tailored shopping experience and improved overall customer experience.
What are some examples of machine learning in e-commerce?
Recommendation engines, like those powering many ecommerce businesses such as Amazon, along with dynamic pricing strategies and chatbots for intuitive customer service, exemplify how machine learning technology enhances customer interactivity and simplifies management processes.
What are best practices for implementing machine learning in e-commerce?
Learn from AI best practices like beginning with a specific goal in mind, leveraging high-quality data for ecommerce machine learning, and consistently tracking to improve performance. Working with expert data scientists helps to ensure machine learning solutions are developed and implemented correctly.
How does machine learning influence e-commerce trends?
These trends, such as personalized marketing, predictive analytics, and automated inventory management, are all driven by machine learning technology. These innovations enable ecommerce businesses to be more competitive and agile in dynamic markets.
What challenges do businesses face when implementing machine learning?
Typical barriers faced in the ecommerce industry are data quality, limited expertise, and integration with legacy systems. Overcoming these challenges is vital for successful ecommerce machine learning adoption.
What ethical considerations should e-commerce businesses keep in mind?
E-commerce companies must prioritize customer privacy and data security, especially as many ecommerce businesses integrate machine learning technology. Ethical considerations not only build consumer trust but also protect online retailers from regulations like GDPR.
What's Your Reaction?
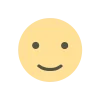
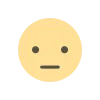
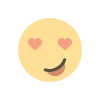
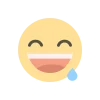
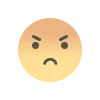
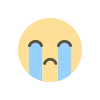
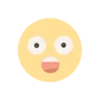